How To Use AI To Create New Products And Services
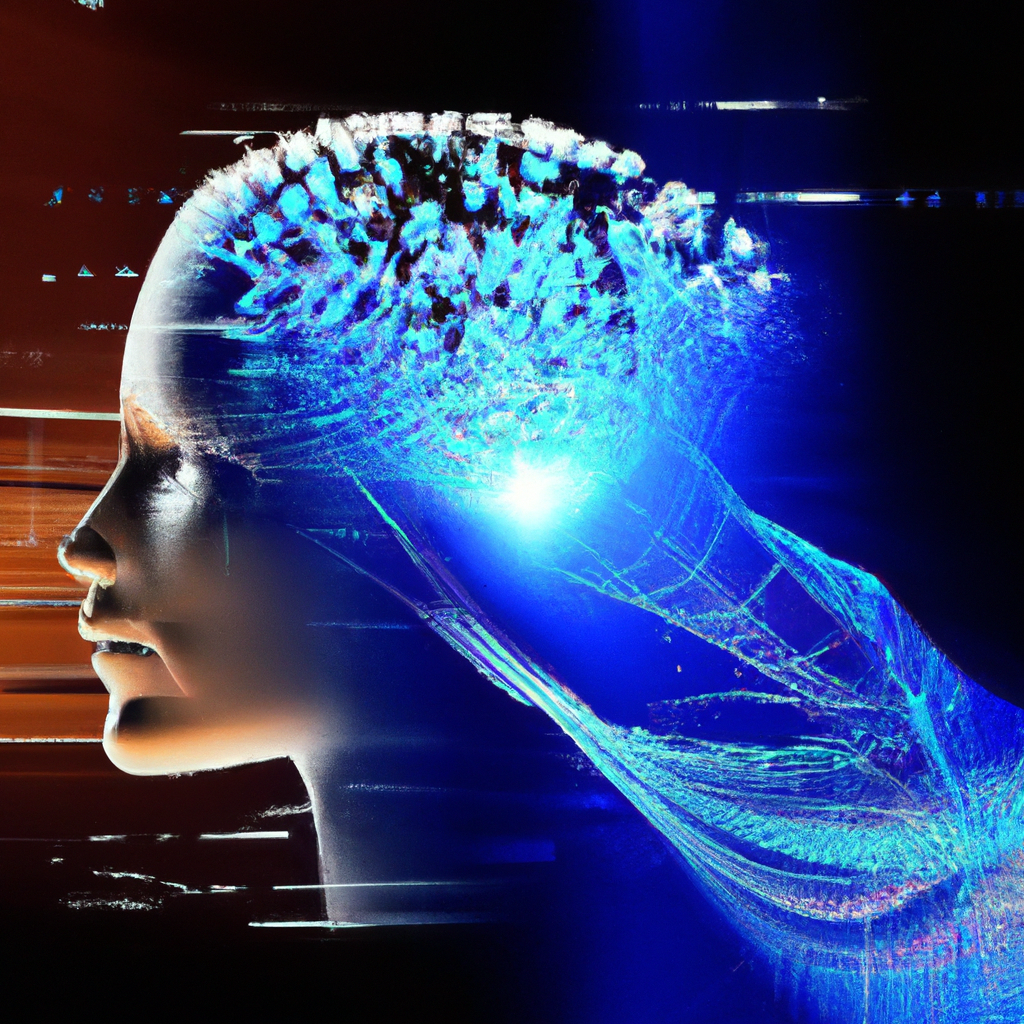
Imagine a world where technology not only catches up with our demands but anticipates them, creating innovative products and services that we didn’t even know we needed. With the advent of artificial intelligence (AI), this dream is becoming a reality. In this article, we will explore the incredible potential of AI in creating new products and services, and how you can harness this cutting-edge technology to unlock untapped opportunities and stay ahead in an ever-evolving market. Get ready to embrace the future of innovation and discover how AI can revolutionize your business.
Understanding AI
Definition of AI
Artificial Intelligence (AI) refers to the ability of computer systems to perform tasks that would typically require human intelligence. It involves the development of algorithms and models that enable machines to learn, reason, and make decisions based on data. AI utilizes techniques such as machine learning, natural language processing, and computer vision to mimic human cognitive functions.
Types of AI
There are various types of AI that can be utilized in product development. Machine learning is a prominent type of AI that involves training models on data to make predictions or decisions. Natural language processing focuses on understanding and deciphering human language, while computer vision enables machines to analyze and interpret visual information. Other AI techniques, such as expert systems, genetic algorithms, and robotic process automation, can also be employed depending on the specific requirements of a product or service.
AI in Product Development
AI has revolutionized product development by enabling the creation of intelligent and innovative solutions. It allows businesses to automate tasks, improve efficiency, and enhance user experiences. By leveraging AI technology, companies can develop products and services that are tailored to meet the evolving needs and preferences of their customers. AI-driven products can range from virtual assistants and recommendation systems to autonomous vehicles and healthcare diagnostics tools. The integration of AI in product development also opens up possibilities for personalized experiences, predictive analytics, and smart automation.
Identifying Opportunities for AI-driven Products and Services
Market Research
Market research is crucial to identify opportunities for AI-driven products and services. It involves gathering insights about market trends, customer preferences, and industry dynamics. By conducting market research, businesses can gain a deeper understanding of their target audience and identify unmet needs or pain points that can be addressed through AI technology. This research helps in assessing market demand and competition to determine the viability and potential success of AI-driven products or services.
Identifying Pain Points
Identifying pain points is a critical step in developing AI-driven solutions. By identifying the challenges and frustrations that customers face, businesses can design products and services that effectively solve these problems. Pain points can range from inefficient processes and lack of personalization to information overload and repetitive tasks. Through interviews, surveys, and data analysis, businesses can collect valuable insights that guide the development of AI-driven solutions that alleviate these pain points and provide value to customers.
Evaluating Feasibility
Before investing resources into AI-driven product development, it is essential to evaluate the feasibility of the proposed solution. Feasibility assessment involves analyzing technical, economic, and operational factors to determine if the product or service can be successfully implemented and sustained. Factors such as data availability and quality, infrastructure requirements, regulatory considerations, and cost-effectiveness need to be taken into account. This evaluation helps in identifying potential risks and challenges, ensuring that the development of AI-driven products or services is viable and aligns with business goals.
Collecting and Preparing Data
Identifying Relevant Data Sources
To develop effective AI models, it is crucial to identify and access relevant data sources. Data sources can include structured data from databases, unstructured data from text documents or social media, and sensor data from IoT devices, among others. Businesses need to determine which data sources are most relevant to their specific AI use cases and evaluate their quality and reliability. Identifying and accessing diverse and representative data sources is essential to ensure the accuracy and generalizability of AI models.
Data Collection Methods
There are various methods for collecting data, depending on the nature of the desired information. Some common methods include surveys, interviews, observational studies, and web scraping. When collecting data, businesses need to define clear objectives, determine sample sizes, and ensure ethical practices to protect user privacy and comply with data regulations. Incorporating data collection methods that provide high-quality and relevant data helps in developing robust and accurate AI models.
Data Cleaning and Preprocessing
Raw data often contains noise, inconsistencies, and missing values that can hinder the performance of AI models. Data cleaning and preprocessing involve transforming and organizing data in a format suitable for analysis. This process includes tasks such as removing duplicates, handling missing data, standardizing variables, and normalizing data distributions. By ensuring data quality and reliability, businesses can improve the accuracy and efficiency of AI models, resulting in more impactful and reliable AI-driven products and services.
Choosing the Right AI Technology
Machine Learning
Machine learning is a powerful AI technology that enables computers to learn and make predictions or decisions without being explicitly programmed. There are different types of machine learning algorithms, including supervised learning, unsupervised learning, and reinforcement learning. Supervised learning involves training models on labeled data to predict future outcomes, while unsupervised learning identifies patterns and relationships in unlabeled data. Reinforcement learning involves training models through trial and error interactions with an environment. Understanding the different types of machine learning algorithms helps businesses choose the most appropriate approach for their AI-driven products or services.
Natural Language Processing
Natural Language Processing (NLP) focuses on enabling computers to understand and interpret human language. It involves tasks such as text classification, sentiment analysis, and language translation. NLP technology allows businesses to develop AI-driven products and services that can understand and respond to natural language inputs, enhancing interactions and providing personalized experiences. Choosing the right NLP techniques and models is essential to ensure accurate and contextually relevant language processing in AI-driven solutions.
Computer Vision
Computer vision enables machines to analyze and interpret visual information, such as images and videos. It involves tasks such as object detection, image recognition, and facial recognition. Computer vision technology has wide-ranging applications, from autonomous vehicles and surveillance systems to medical diagnostics and augmented reality. Choosing suitable computer vision algorithms and models is crucial for developing accurate and reliable AI-driven products and services that can interpret and process visual data effectively.
Other AI Techniques
Besides machine learning, natural language processing, and computer vision, there are various other AI techniques that can be employed in product development. Expert systems utilize knowledge from human experts to assist in decision-making, while genetic algorithms mimic the process of natural selection to optimize solutions. Robotic process automation automates repetitive tasks, improving efficiency and productivity. By exploring and understanding other AI techniques, businesses can identify additional opportunities for creating innovative and intelligent products or services.
Training AI Models
Supervised Learning
Supervised learning is a machine learning technique that involves training models on labeled data. Labeled data consists of inputs and corresponding outputs, allowing the model to learn patterns and make predictions based on new inputs. This approach is suitable when the desired outcome is known and sufficient labeled data is available. Through iterative training and optimization, businesses can develop AI models that accurately predict outcomes or classify new inputs, enabling the creation of intelligent products or services.
Unsupervised Learning
Unsupervised learning involves training models on unlabeled data to uncover patterns, relationships, or groups in the data. This approach is useful when the desired outcome is unknown or when exploring the structure of the data. Unsupervised learning algorithms, such as clustering and dimensionality reduction, help in identifying hidden patterns or similarities within the data. By utilizing unsupervised learning techniques, businesses can gain insights from large datasets and discover valuable information that can drive product development decisions.
Reinforcement Learning
Reinforcement learning involves training models through trial and error interactions with an environment. The model learns by receiving feedback or rewards for its actions and continuously improves its behavior to maximize the rewards. This learning approach is suitable for scenarios where the optimal solution is not predefined, and the model needs to explore different actions to achieve a specific goal. Reinforcement learning has been successfully applied in areas such as game playing, robotics, and autonomous systems.
Acquiring Labeled Data
Training AI models requires labeled data, which can be obtained through various methods. Businesses can acquire labeled data through manual annotation by domain experts or by crowd-sourcing data labeling tasks. Organizations may also leverage existing datasets that are publicly available or purchase labeled data from third-party providers. Acquiring high-quality labeled data is crucial for training accurate and reliable AI models, ensuring the success of AI-driven products and services.
Integrating AI into Products and Services
Defining AI Product Requirements
When integrating AI into products and services, it is essential to define clear requirements and objectives. This involves identifying the specific tasks or functionalities that AI will enhance or automate. Defining product requirements helps in aligning AI capabilities with business goals, ensuring that the integration of AI technology brings value and addresses customers’ needs. Clear requirements also guide the development process and facilitate effective collaboration between AI experts, designers, and developers.
Identifying AI Use Cases
Identifying AI use cases involves determining the areas where AI can provide the most value and impact. By identifying use cases, businesses can focus their efforts on developing AI-driven products or services that solve specific problems or improve existing processes. Use cases can range from personalized recommendations and intelligent chatbots to predictive maintenance and fraud detection. By pinpointing the most relevant use cases, businesses can prioritize their AI integration efforts and maximize the potential benefits.
Designing User Interfaces
Designing user interfaces (UI) that effectively incorporate AI capabilities is crucial for a seamless user experience. AI should be integrated in a way that is intuitive and enhances the usability of the product or service. User interfaces should be designed to effectively communicate AI-driven features and provide users with control and transparency. By considering user needs, preferences, and expectations, businesses can develop AI-driven products and services with user-friendly interfaces that drive engagement and adoption.
Developing AI Solutions
Selecting the Right Development Tools
Developing AI solutions requires the selection of appropriate tools and frameworks. There are numerous AI development platforms and libraries available that provide pre-built components and algorithms, facilitating the development process. Choosing the right development tools depends on the specific AI techniques and requirements of the product or service. Tools such as TensorFlow, PyTorch, and scikit-learn offer comprehensive support for machine learning, while libraries like NLTK and spaCy provide NLP capabilities. By selecting the right development tools, businesses can streamline the development process and improve the efficiency of AI solutions.
Implementing AI Algorithms
Implementing AI algorithms involves translating the chosen AI techniques into code. This step requires expertise in programming languages such as Python, Java, or R, depending on the selected tools and frameworks. AI algorithms need to be implemented accurately, ensuring their functionality and compatibility with the chosen development environment. During implementation, it is crucial to conduct thorough testing and validation to identify and resolve any issues or inefficiencies and optimize the performance of AI solutions.
Testing and Debugging
Testing and debugging AI solutions is a critical step in ensuring their reliability and accuracy. Robust testing involves evaluating the performance of AI models against different datasets, including both training and unseen data. This process includes techniques such as cross-validation, A/B testing, and stress testing to assess the model’s behavior and identify any areas for improvement. Debugging involves identifying and addressing any errors, inconsistencies, or performance issues that arise during testing. Rigorous testing and debugging help in producing AI solutions that deliver consistent and reliable results.
Ensuring Data Privacy and Security
Anonymizing Personally Identifiable Information
Data privacy is paramount when handling sensitive data in AI-driven products and services. Anonymizing personally identifiable information (PII) is an important practice to protect user privacy. PII refers to any information that can be used to identify an individual, such as names, addresses, or social security numbers. By implementing techniques such as tokenization, data masking, or differential privacy, businesses can ensure that PII is not exposed in AI processes while still retaining the utility of the data.
Implementing Encryption
Encryption plays a critical role in securing data in transit and at rest. It involves converting data into an unreadable format using cryptographic algorithms, making it unintelligible to unauthorized users. Implementing encryption techniques, such as secure socket layer (SSL) or transport layer security (TLS), protects data as it is transmitted between different systems or when stored in databases. Encryption is an essential component of data security practices in AI-driven products and services.
Implementing Access Controls
Access controls help in ensuring that only authorized personnel can access and manipulate data in AI systems. Implementing access controls involves defining user roles, permissions, and authentication mechanisms to restrict access to sensitive data and functionalities. Role-based access control (RBAC), multi-factor authentication (MFA), and secure APIs are examples of access control mechanisms that can be implemented to ensure data security. By enforcing strict access controls, businesses can mitigate the risk of unauthorized access or data breaches in AI-driven products and services.
Monitoring and Improving AI Models
Performance Monitoring
Monitoring the performance of AI models is essential to ensure their accuracy and effectiveness over time. Performance monitoring involves tracking key metrics, such as predictive accuracy, response time, and resource utilization. By continuously monitoring these metrics, businesses can identify any performance degradation or anomalies and take proactive measures to maintain or improve the performance of AI models. Performance monitoring also helps in identifying the need for retraining or fine-tuning models as new data becomes available.
Continuous Learning
Continuous learning is an ongoing process of updating AI models with new data to improve their performance and adaptability. By feeding new data into the models, businesses can ensure that the models remain up-to-date and relevant. Continuous learning can involve techniques such as online learning, where the model is updated in real-time as new data arrives, or periodic retraining, where the model is retrained at regular intervals to incorporate new information. Continuous learning enables AI-driven products and services to evolve and improve over time.
Addressing Bias and Ethical Concerns
AI models can be susceptible to biases that reflect the biases present in the data used for training. Addressing bias and ethical concerns is crucial to ensure fairness and equality in AI-driven products and services. It involves evaluating and mitigating biases in data, refining algorithms to minimize bias amplification, and conducting thorough testing to ensure fairness across different demographic groups. By prioritizing ethical considerations and addressing biases, businesses can build AI models that promote inclusivity and avoid unintended discriminatory outcomes.
Evaluating the Success of AI-driven Products and Services
Defining Key Performance Indicators
To evaluate the success of AI-driven products and services, businesses need to define key performance indicators (KPIs). KPIs are quantifiable metrics that measure the performance or effectiveness of a product or service. Examples of KPIs for AI-driven products can include user engagement, conversion rates, customer satisfaction, or cost savings. By establishing clear KPIs, businesses can track the impact and value generated by AI and assess the overall success and ROI of their AI-driven products or services.
User Feedback and Satisfaction
User feedback and satisfaction are critical factors in evaluating the success of AI-driven products and services. Gathering user feedback through surveys, interviews, or reviews helps businesses understand user experiences, identify areas for improvement, and validate the value delivered by AI features. Analyzing user satisfaction scores, customer ratings, and user engagement metrics provides insights into the overall reception and adoption of AI-driven products or services. User feedback and satisfaction are valuable indicators of the success and impact of AI integration.
Continuous Improvement
Continuous improvement is an essential aspect of evaluating and enhancing AI-driven products and services. It involves analyzing performance data, user feedback, and market trends to identify opportunities for optimization and innovation. By continuously iterating on AI models, algorithms, and user interfaces, businesses can enhance the functionality, accuracy, and value of their products or services. Continuous improvement ensures that AI-driven solutions remain competitive, adaptable, and aligned with evolving customer needs.
In conclusion, AI offers immense potential for creating new products and services that revolutionize industries and enhance user experiences. By understanding the various aspects of AI, identifying opportunities, collecting and preparing data, choosing the right AI technology, training models, integrating AI into products, ensuring data privacy and security, monitoring and improving models, and evaluating success, businesses can effectively harness AI to drive innovation and deliver impactful AI-driven products and services.