The Latest AI Research
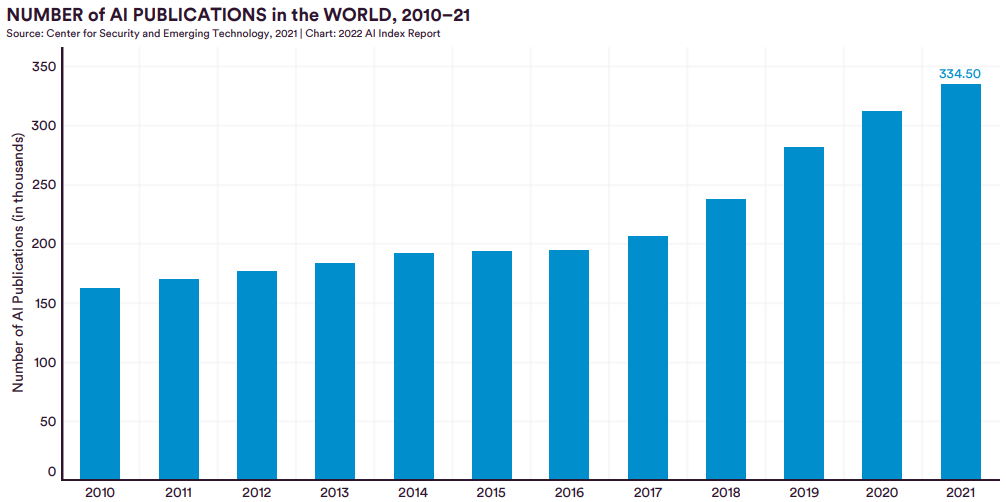
Imagine a world where machines can think, learn, and make decisions just like humans. Incredible, isn’t it? Well, the latest advancements in artificial intelligence (AI) research are bringing us closer to this reality than ever before. From self-driving cars to virtual assistants, AI is revolutionizing our daily lives in unimaginable ways. In this article, we will explore the cutting-edge discoveries and breakthroughs in the field of AI, providing you with a glimpse into the minds of these intelligent machines and the incredible potential they hold for the future.
This image is property of cdn11.bigcommerce.com.
1. Machine Learning
Machine learning is a branch of artificial intelligence that focuses on developing algorithms and models that allow computers to learn from and make predictions or decisions based on data without explicit programming. There are several subfields within machine learning, each with its own unique applications and techniques. In this article, we will explore three prominent areas of machine learning: reinforcement learning, deep learning, and generative adversarial networks.
1.1 Reinforcement Learning
Reinforcement learning is a type of machine learning that involves training an agent to make decisions or take actions in an environment in order to maximize a reward. The agent learns through a process of trial and error, exploring different actions and learning from the feedback it receives. This approach has been successfully applied to a wide range of tasks, including playing games, autonomous navigation, and robotics.
One notable application of reinforcement learning is in the field of healthcare, where it has been used to develop algorithms that can optimize treatment plans for patients with chronic diseases. By continuously learning from patient data and adjusting treatment strategies, these algorithms can improve patient outcomes and reduce healthcare costs.
1.2 Deep Learning
Deep learning is a subfield of machine learning that focuses on training artificial neural networks with multiple layers of interconnected nodes, or “neurons.” These networks are inspired by the structure and function of the human brain and can learn to recognize patterns and make predictions from large amounts of data.
Deep learning has revolutionized many areas of AI, including natural language processing, computer vision, and speech recognition. For example, deep learning models have enabled significant advancements in image recognition, allowing computers to identify and classify objects in images with impressive accuracy.
1.3 Generative Adversarial Networks
Generative adversarial networks (GANs) are a type of machine learning model that consists of two components: a generator and a discriminator. The generator attempts to create synthetic data that resembles the real data it is trained on, while the discriminator tries to distinguish between the real and synthetic data.
GANs have been highly successful in generating realistic images, music, and even text. They have also been used to enhance data augmentation techniques, which can improve the performance of machine learning models by increasing the diversity and quantity of training data.
2. Natural Language Processing
Natural language processing (NLP) involves teaching computers to understand, interpret, and generate human language. This field combines techniques from both linguistics and computer science to enable machines to communicate with humans in a more natural and meaningful way. Three important areas of NLP are sentiment analysis, chatbots, and language translation.
2.1 Sentiment Analysis
Sentiment analysis, also known as opinion mining, is the process of determining the sentiment or emotional tone expressed in a piece of text. This technique is commonly used to analyze social media posts, customer reviews, and other forms of user-generated content to gauge public opinion or customer satisfaction.
By utilizing sentiment analysis, companies can gain valuable insights into customer preferences and opinions, allowing them to make data-driven decisions and improve their products or services. This can be particularly useful in fields such as marketing, customer service, and brand management.
2.2 Chatbots
Chatbots are computer programs designed to simulate human conversation and provide automated responses. They are often used in customer service, where they can handle routine queries, provide information, and assist customers with basic tasks. Chatbots utilize techniques from NLP to understand and generate human language, enabling them to communicate effectively with users.
Chatbots have the potential to streamline customer interactions, reduce response times, and enhance user experiences. As advancements in NLP continue to improve the accuracy and naturalness of chatbot conversations, they are becoming increasingly prevalent in various industries, from e-commerce to healthcare.
2.3 Language Translation
Language translation is the process of translating text or speech from one language to another. With the help of AI and NLP techniques, machine translation systems have made significant progress in recent years. These systems can now accurately translate between multiple languages, enabling people from different linguistic backgrounds to communicate effectively.
Language translation has numerous applications, from facilitating international business and travel to enabling cross-cultural communication and information sharing. AI-powered translation tools have the potential to break down language barriers and promote global collaboration and understanding.
This image is property of miro.medium.com.
3. Computer Vision
Computer vision is a field of AI that focuses on developing algorithms and systems that enable computers to analyze, understand, and interpret visual information from images or videos. This technology aims to replicate and enhance the human visual system, enabling computers to recognize objects, faces, and gestures, and extract meaningful information from visual data.
3.1 Object Detection and Recognition
Object detection and recognition involve identifying and locating specific objects within images or videos. This technology has numerous applications, ranging from autonomous driving and surveillance to augmented reality and image search. By accurately detecting and recognizing objects, computers can perform tasks such as automated inventory management, facial recognition for security purposes, and even identifying potential hazards in industrial settings.
3.2 Image Segmentation
Image segmentation refers to the process of dividing an image into multiple regions or segments based on certain attributes or features. This technique allows computers to understand the different elements within an image and analyze them separately. For example, image segmentation can be used to identify and extract specific objects or regions of interest in medical imaging, satellite imagery, and remote sensing.
Image segmentation is a critical component of various computer vision applications, such as autonomous navigation, image editing, and medical diagnostics. By accurately segmenting images, computers can gain a deeper understanding of the visual information and make more informed decisions.
3.3 Facial Recognition
Facial recognition technology involves identifying and verifying an individual’s identity by analyzing and comparing their facial features. This technology has gained significant attention in recent years due to its applications in security, surveillance, and authentication systems.
Facial recognition systems can be used to enhance security measures in various domains, including airports, border control, and access control systems. They can also be used for personal identification and authentication, enabling secure and convenient access to devices, applications, and online services.
4. Robotics and Automation
Robotics and automation involve the integration of AI technologies with physical systems and devices to automate tasks and enhance efficiency. These technologies have the potential to revolutionize industries and improve various aspects of daily life. Three important areas of robotics and automation are autonomous vehicles, industrial automation, and humanoid robots.
4.1 Autonomous Vehicles
Autonomous vehicles, also known as self-driving cars, are vehicles capable of operating without human intervention. These vehicles use a combination of sensors, computer vision, and machine learning algorithms to navigate roads, detect obstacles, and make decisions in real-time.
The development of autonomous vehicles has the potential to transform transportation systems, making them safer, more efficient, and more accessible. Self-driving cars can reduce traffic congestion, lower the number of accidents caused by human error, and improve mobility for individuals with disabilities or limited access to transportation.
4.2 Industrial Automation
Industrial automation involves using AI and robotics to automate manufacturing processes and improve productivity and efficiency in industrial settings. This technology can be applied to numerous tasks, including assembly line operations, quality control, and material handling.
By automating repetitive and physically demanding tasks, industrial automation can enhance worker safety, reduce errors and defects, and increase production output. It allows companies to streamline their operations, reduce costs, and stay competitive in the global market.
4.3 Humanoid Robots
Humanoid robots are robots designed to resemble and interact with humans in a natural way. These robots incorporate AI technologies such as computer vision, natural language processing, and machine learning to understand and respond to human commands, gestures, and emotions.
Humanoid robots have the potential to assist humans in various domains, ranging from healthcare and elderly care to education and entertainment. They can perform tasks such as providing companionship to elderly individuals, assisting in rehabilitation therapies, and even teaching complex subjects to students.
This image is property of www.analyticsinsight.net.
5. Healthcare Applications
AI has the potential to revolutionize healthcare by improving diagnostics, treatment strategies, and patient care. Three significant applications of AI in healthcare are disease diagnosis, drug discovery, and remote patient monitoring.
5.1 Disease Diagnosis
AI algorithms can analyze medical images, patient data, and electronic health records to assist healthcare professionals in diagnosing diseases and conditions with high accuracy and efficiency. Machine learning techniques can be trained to detect patterns and abnormalities in medical images, aiding in the early detection of diseases such as cancer, cardiovascular conditions, and neurological disorders.
By providing accurate and timely diagnoses, AI-powered systems can improve patient outcomes, reduce the need for invasive procedures, and enable more personalized treatment plans. They can assist healthcare professionals in making informed decisions and delivering better quality care.
5.2 Drug Discovery
The process of discovering and developing new drugs is time-consuming, expensive, and often inefficient. AI technologies, such as machine learning and deep learning, can accelerate the drug discovery process by analyzing vast amounts of data, predicting drug-target interactions, and optimizing chemical compounds.
AI-powered drug discovery has the potential to identify promising drug candidates, reduce the cost and time required for clinical trials, and facilitate the development of personalized medicine. It can also aid in the repurposing of existing drugs for new therapeutic applications, providing new treatment options for patients.
5.3 Remote Patient Monitoring
Remote patient monitoring involves collecting and analyzing patient data, such as vital signs, symptoms, and medication adherence, from a distance. AI algorithms can leverage this data to detect early warning signs of deterioration, predict disease progression, and provide personalized recommendations for patients.
By enabling healthcare providers to monitor patients remotely, AI-powered systems can improve patient access to care, reduce hospital readmissions, and enhance patient outcomes. Remote patient monitoring is particularly valuable for individuals with chronic conditions, elderly patients, and those living in remote or underserved areas.
6. Ethical Considerations
As AI technologies continue to advance and become more integrated into our daily lives, it is crucial to consider the ethical implications and potential risks associated with their use. Three key ethical considerations in AI are bias in AI systems, privacy and data protection, and algorithmic accountability.
6.1 Bias in AI Systems
AI systems are only as reliable and unbiased as the data they are trained on. If the training data contains biases, these biases can be amplified and perpetuated by the AI system, leading to discriminatory outcomes. It is essential to ensure that AI systems are trained on diverse and representative datasets to mitigate the risk of bias.
Addressing bias in AI systems requires a multidisciplinary approach, involving experts from various fields, including ethics, sociology, and data science. Companies and organizations also need to establish ethical guidelines and practices for developing and deploying AI systems, and be transparent about the limitations and biases of these technologies.
6.2 Privacy and Data Protection
AI technologies rely on vast amounts of data to learn and make predictions. This data often includes personal and sensitive information, raising concerns about privacy and data protection. It is essential to establish robust data governance frameworks and ensure that individuals have control over their data and how it is used.
Companies and organizations must adhere to strict data protection regulations and implement measures to secure data against unauthorized access or misuse. They should also be transparent about their data collection and usage practices, and obtain informed consent from individuals before collecting or processing their data.
6.3 Algorithmic Accountability
Algorithmic accountability refers to the responsibility of developers and users of AI systems to ensure that these systems are fair, transparent, and accountable for their actions. AI algorithms can be complex and difficult to understand, leading to concerns about their decision-making processes and potential biases.
To address algorithmic accountability, there is a need for explainable AI techniques that can provide insights into how AI systems arrive at their decisions. Regulatory frameworks and industry standards can also play a crucial role in holding developers and users accountable for the ethical use of AI technologies.
This image is property of about.fb.com.
7. AI in Finance
AI technologies have had a significant impact on the financial industry, enabling organizations to automate tasks, improve risk management, and enhance customer experiences. Three important applications of AI in finance are fraud detection, automated trading, and risk assessment.
7.1 Fraud Detection
AI-powered fraud detection systems can analyze vast amounts of financial data, transaction records, and customer behavior to identify and flag potentially fraudulent activities. Machine learning algorithms can learn patterns of fraudulent behavior and adapt to new fraud techniques, helping financial institutions prevent and respond to fraudulent transactions.
By detecting and mitigating fraud, AI systems can protect the interests of customers, reduce financial losses, and maintain the integrity of financial systems. They can also help streamline the investigation process, enabling faster resolution of fraudulent cases.
7.2 Automated Trading
Automated trading, also known as algorithmic trading, involves using AI algorithms and high-frequency trading systems to execute trades in financial markets. These systems can analyze real-time market data, identify patterns, and make trading decisions in milliseconds, without human intervention.
Automated trading can enhance market liquidity, increase trading efficiency, and reduce transaction costs. It allows financial firms to exploit market opportunities and respond to market changes quickly. However, it also raises concerns about the potential for market manipulation and the need for robust regulation and oversight.
7.3 Risk Assessment
AI technologies can assist financial institutions in assessing and managing risks by analyzing market data, credit scores, and economic indicators. Machine learning algorithms can learn from historical data and identify patterns and trends that can help predict future market dynamics and potential risks.
By providing accurate risk assessments, AI-powered systems can help financial institutions make informed decisions, optimize their portfolios, and improve profitability. They can also enable regulators and policymakers to monitor and mitigate systemic risks in the financial system.
8. AI in Education
AI has the potential to transform the field of education by personalizing learning experiences, improving instructional strategies, and enhancing student outcomes. Three significant applications of AI in education are intelligent tutoring systems, personalized learning, and automated grading.
8.1 Intelligent Tutoring Systems
Intelligent tutoring systems (ITS) are computer-based programs that provide personalized instruction and feedback to students. These systems utilize AI techniques to assess the knowledge and skills of individual students and tailor instruction to their needs and learning styles.
ITS can simulate one-on-one tutoring, providing students with personalized support and guidance. They can adapt the pace and content of instruction based on the student’s performance, helping them grasp difficult concepts and master new skills more effectively.
8.2 Personalized Learning
Personalized learning involves tailoring educational content, activities, and assessments to the specific needs and preferences of individual students. AI technologies can analyze data on student progress, learning styles, and preferences to create adaptive learning environments that optimize engagement and achievement.
By providing personalized learning experiences, AI technologies can cater to the diverse learning needs of students, accommodate different learning paces, and ensure that each student receives the support they require. Personalized learning has the potential to enhance student motivation, increase learning outcomes, and improve equity in education.
8.3 Automated Grading
Grading and assessment are time-consuming tasks for teachers. AI technologies can automate the grading process by analyzing student responses and applying predefined grading criteria. Automated grading systems can provide immediate feedback to students, enabling them to track their progress and identify areas for improvement.
Automated grading can save teachers time, allowing them to focus on instructional activities, provide timely feedback to students, and focus on individualized instruction. However, it also raises concerns about the reliability and fairness of grading algorithms and the potential for algorithmic bias.
This image is property of s.yimg.com.
9. Quantum Computing and AI
Quantum computing is a rapidly developing field that combines principles from quantum physics and computer science to develop powerful computational systems. When combined with AI techniques, quantum computing has the potential to achieve breakthroughs in areas such as quantum machine learning, quantum neural networks, and quantum algorithms.
9.1 Quantum Machine Learning
Quantum machine learning explores the use of quantum computing to enhance machine learning algorithms and models. By leveraging the unique properties of quantum systems, such as superposition and entanglement, quantum machine learning algorithms can potentially solve problems that are currently intractable for classical computers.
Quantum machine learning has the potential to accelerate the training and optimization of machine learning models, improve pattern recognition capabilities, and enable more accurate predictions. However, the field is still in its early stages, and significant challenges, such as noise and error correction, need to be overcome to realize its full potential.
9.2 Quantum Neural Networks
Quantum neural networks (QNNs) are a type of artificial neural network designed to operate on quantum computers. QNNs aim to leverage the capabilities of quantum systems to enhance the learning and decision-making capabilities of neural networks.
QNNs can potentially solve complex optimization problems, perform efficient data compression, and simulate quantum systems. They offer a promising avenue for developing AI applications that can leverage the power of quantum computing to address challenges that are currently beyond the capabilities of classical computers.
9.3 Quantum Algorithms
Quantum algorithms are computational procedures specifically designed to be executed on quantum computers. These algorithms can utilize the unique properties of quantum systems, such as superposition and interference, to solve problems more efficiently than classical algorithms.
Quantum algorithms have the potential to enhance various AI applications, including optimization, simulation, and machine learning. They can offer exponential speedup in solving certain computational problems, leading to breakthroughs in areas such as cryptography, optimization, and data analysis.
10. AI and the Internet of Things
The Internet of Things (IoT) refers to the network of interconnected devices and sensors that collect and exchange data. By combining AI technologies with IoT devices, it is possible to create intelligent systems that can analyze and interpret the vast amounts of data generated by these devices. Three important applications of AI in IoT are smart homes, industrial IoT, and wearable devices.
10.1 Smart Homes
AI technologies can transform traditional homes into smart homes by integrating IoT devices and sensors with intelligent systems. Smart homes can automate various tasks, such as controlling lighting, heating, and security systems, based on user preferences and environmental conditions.
By utilizing AI, smart homes can learn from user behavior and adapt their functions and settings to optimize energy usage, improve comfort, and enhance security. They can also enable remote monitoring and control, allowing users to manage their homes from anywhere.
10.2 Industrial IoT
Industrial IoT involves integrating AI technologies with industrial systems and processes to enhance efficiency, productivity, and safety. AI-powered industrial IoT systems can collect and analyze real-time data from sensors, machines, and production lines to optimize operations, detect anomalies, and predict maintenance needs.
By enabling predictive maintenance, real-time monitoring, and adaptive control, industrial IoT systems can reduce downtime, improve asset utilization, and minimize the risk of accidents. They can transform traditional factories into intelligent, data-driven environments where machines and humans collaborate to achieve optimal performance.
10.3 Wearable Devices
Wearable devices, such as smartwatches and fitness trackers, have become increasingly popular in recent years. These devices collect various types of data, including biometric data, activity levels, and sleep patterns. By integrating AI technologies, wearable devices can analyze this data and provide personalized insights and recommendations to users.
AI-powered wearable devices can help individuals monitor and improve their health and well-being, track fitness goals, and detect early signs of health issues. They can also enable remote patient monitoring, allowing healthcare professionals to monitor patients’ health status and provide timely interventions.
In conclusion, AI technologies have transformed various fields, from healthcare and finance to education and robotics. From machine learning and natural language processing to computer vision and robotics, AI has the potential to revolutionize industries, enhance decision-making processes, and improve the quality of our lives. However, it is crucial to consider the ethical implications and potential risks associated with AI and to ensure that these technologies are developed and deployed responsibly, with the utmost consideration for privacy, fairness, and accountability.