The Impact Of AI On The Environment
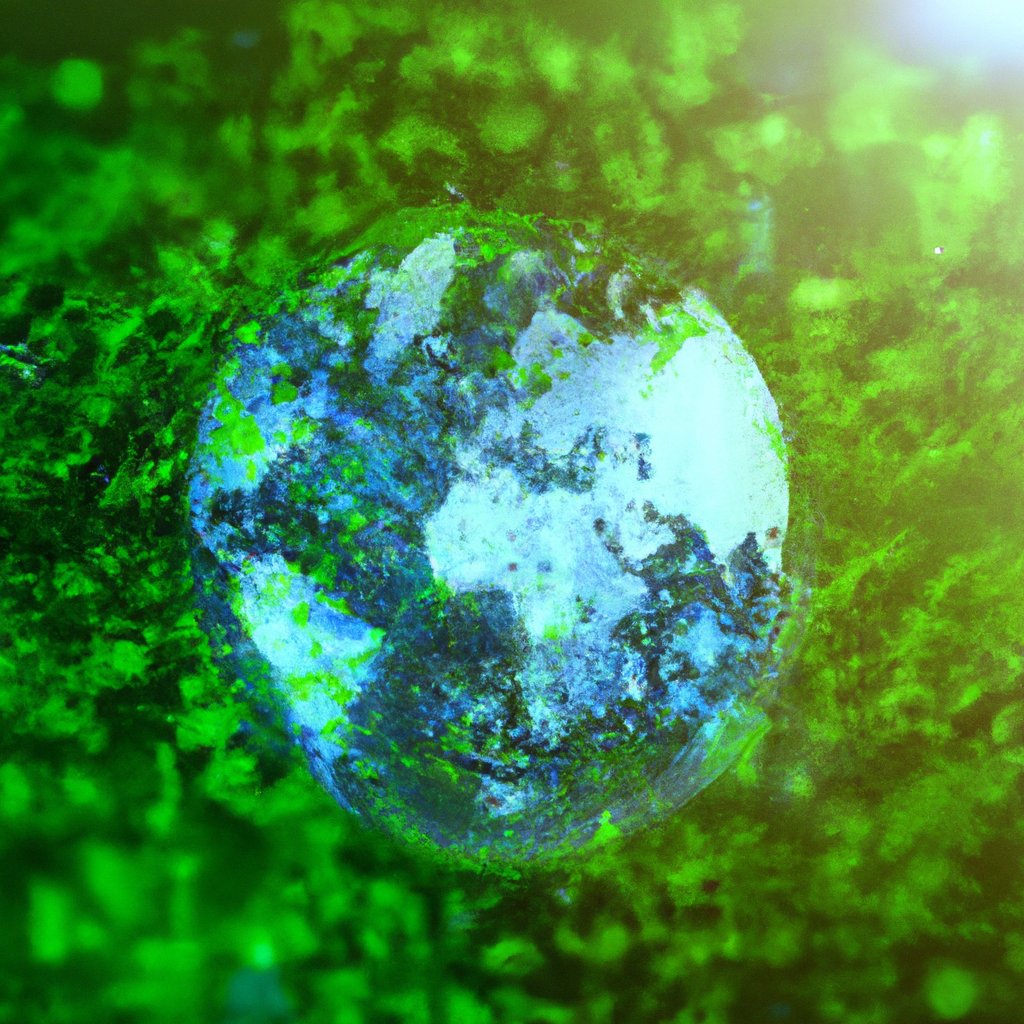
Have you ever stopped to think about the potential consequences of artificial intelligence (AI) on the environment? As we step into the era of advanced technology, it becomes crucial to understand how AI can influence the world around us. From energy consumption to carbon footprint, AI has the power to reshape our environmental landscape. In this article, we will explore the impact of AI on the environment and the potential challenges and solutions that lie ahead. So, buckle up and let’s delve into the fascinating world where cutting-edge technology meets sustainability.
1. Energy Consumption
1.1 Data Centers
Data centers play a crucial role in supporting the rapid growth of AI technology. These centers require substantial amounts of energy to power and cool the servers and equipment they house. The electricity consumption of data centers is a significant contributor to the overall energy consumption associated with AI. It is estimated that data centers globally consume about 1% of the global electricity supply. However, advancements in energy-efficient hardware and cooling technologies are being made to address these concerns.
1.2 Training AI Models
Training AI models requires significant computational power, which translates to high energy consumption. The complex algorithms and vast datasets used in training AI models demand extensive processing capabilities, which in turn require substantial energy inputs. It is important to explore energy-efficient methodologies and algorithms to reduce the environmental impact of training AI models without compromising on their performance.
1.3 AI-powered Devices
From smartphones to smart home devices, the proliferation of AI-powered devices has led to an increase in energy consumption. These devices often rely on constant connectivity and real-time data processing, which can contribute to higher energy usage. However, the industry is actively working towards developing energy-efficient AI chips and optimizing algorithms to minimize energy consumption while maintaining functionality.
2. Carbon Emissions
2.1 Data Centers
The energy consumed by data centers not only contributes to increased energy consumption but also leads to significant carbon emissions. The generation of electricity from fossil fuels, which is still prevalent in many regions, results in the release of greenhouse gases. Green data center initiatives, such as utilizing renewable energy sources and adopting more efficient cooling systems, are being implemented to mitigate carbon emissions.
2.2 Manufacturing AI Hardware
The production of AI hardware, including chips and sensors, requires resource-intensive processes that contribute to carbon emissions. The extraction and manufacturing of components, along with the assembly and transportation of these devices, have significant environmental implications. Organizations are striving to minimize the carbon footprint of AI hardware production by adopting sustainable manufacturing practices, reducing waste, and exploring alternative materials.
2.3 End-User Energy Use
The energy consumption of AI-powered devices at the end-user level also contributes to carbon emissions. As more individuals leverage AI technologies in their daily lives, the collective energy usage increases. However, encouraging energy-efficient behaviors among users and designing devices with efficient power management capabilities can help reduce the environmental impact of end-user energy consumption.
2.4 Electronic Waste
The rapid pace of technological advancements in AI leads to a constant influx of new devices in the market, resulting in electronic waste. Improper disposal and recycling of AI devices can have severe consequences for the environment. E-waste contains hazardous materials, such as lead, mercury, and cadmium, which can contaminate soil and water if not handled properly. Implementing effective e-waste management systems, including recycling and proper disposal, is vital for minimizing the environmental impact of AI technology.
3. Resource Extraction
3.1 Rare Earth Minerals
AI hardware components, such as magnets and capacitors, often rely on rare earth minerals for their functionality. The extraction of these minerals can have detrimental effects on the environment, including habitat destruction, water pollution, and soil degradation. It is essential to develop sustainable practices for rare earth mineral extraction and explore alternatives to reduce the dependency on these finite resources.
3.2 Water Consumption
Water is a vital resource in AI-related processes, including cooling systems in data centers and manufacturing operations. The substantial water requirements of data centers can strain local water supplies, especially in regions facing water scarcity. Implementing water-efficient cooling technologies and utilizing recycled water can help minimize the environmental impact of water consumption in AI operations.
4. Environmental Impact of Data Collection
4.1 Privacy Concerns
The collection and processing of massive amounts of data for AI applications raise concerns regarding privacy. As AI algorithms rely heavily on data, there is a need to address privacy concerns associated with data collection and storage. Privacy regulations and data protection practices are crucial to ensure that personal information is handled responsibly and securely.
4.2 Data Storage Needs
The storage of vast amounts of data for AI applications requires significant server infrastructure and energy consumption. As data storage requirements increase, the environmental impact of maintaining these storage systems also grows. Adopting efficient data storage practices, including data deduplication, compression, and cloud-based storage solutions, can help reduce the carbon footprint associated with data storage.
5. Impact on Transportation and Logistics
5.1 Autonomous Vehicles
AI has the potential to revolutionize transportation through the development of autonomous vehicles. These vehicles offer the promise of increased safety and efficiency on the roads. By optimizing routes and reducing congestion, AI-powered autonomous vehicles have the potential to decrease fuel consumption and emissions. However, the manufacturing process of these vehicles and the associated infrastructure need to consider sustainability and employ renewable energy sources to achieve a truly environmentally friendly transportation system.
5.2 Smart Traffic Management
AI can also contribute to more efficient traffic management systems. By analyzing real-time data, AI algorithms can optimize traffic flow, reducing congestion and idling time. This optimization can have a positive impact on fuel consumption and emissions associated with transportation. Smart traffic management systems can also provide valuable information to drivers, enabling them to make more eco-friendly travel decisions.
6. Sustainable and Green AI
6.1 AI for Environmental Monitoring
AI can be harnessed for environmental monitoring, helping to assess ecological conditions, track biodiversity, and detect environmental changes or pollution. By utilizing AI algorithms to process and analyze large amounts of data collected from remote sensors, satellites, and drones, scientists and policymakers can make informed decisions for environmental conservation and sustainable resource management.
6.2 Energy Efficiency Optimization
AI can play a significant role in optimizing energy efficiency across various sectors, including industries and buildings. Machine learning algorithms can analyze energy consumption patterns and identify opportunities for improvement, helping to reduce energy waste and lower carbon emissions. AI-driven energy management systems can dynamically adjust energy usage based on demand and generate insights for energy efficiency upgrades.
6.3 Recycling and Waste Management
AI technologies can aid in improving recycling and waste management processes. Machine learning algorithms can facilitate sorting and categorizing recyclable materials, enhancing the efficiency of recycling operations. AI-powered systems can also optimize waste collection routes, reducing fuel consumption and emissions associated with waste management. Furthermore, AI can help identify patterns in waste generation and inform policies for waste reduction and sustainable waste management practices.
7. Regulatory Challenges
7.1 Balancing Innovation and Environment
The rapid pace of AI innovation raises challenges in balancing the need for technological advancements with the environmental impact. Regulations and policies play a vital role in ensuring that AI development takes into account the environmental consequences. Governments and regulatory bodies need to collaborate with AI developers, industry experts, and environmental organizations to create frameworks that encourage sustainable AI practices without stifling innovation.
7.2 Data Privacy and Security
As AI technologies rely heavily on data, ensuring data privacy and security is of utmost importance. Striking a balance between data access and protection is crucial to maintain user trust and safeguard sensitive information. Governments and organizations need to establish robust data privacy regulations, along with implementing encryption and cybersecurity measures, to address the concerns associated with the collection and use of personal data for AI applications.
8. AI Solutions for Environmental Challenges
8.1 Climate Change Prediction and Mitigation
AI can aid in predicting and mitigating the impacts of climate change. Machine learning algorithms can analyze large-scale climate data to identify patterns and make accurate predictions about future climatic conditions. AI can also optimize energy generation and distribution systems, enable smart grid management, and support the integration of renewable energy sources to combat climate change.
8.2 Natural Disaster Management
AI technologies can enhance early warning systems and disaster response capabilities. By analyzing historical data and real-time information, AI algorithms can improve the accuracy of predicting natural disasters and mitigate their impact through effective planning and resource allocation. AI-powered systems can also support emergency response operations by providing real-time situational awareness and aiding in decision-making processes.
8.3 Biodiversity Conservation
AI can contribute to biodiversity conservation through various applications. By analyzing ecological data, AI algorithms can monitor wildlife populations, detect illegal activities such as poaching or deforestation, and provide insights for habitat restoration and conservation efforts. AI-powered systems can also help identify invasive species and support the development of strategies to mitigate their impact on ecosystems.
9. Role of AI in Renewable Energy
9.1 Smart Grids
AI can optimize the functioning of smart grids by analyzing data from multiple sources, including energy consumption patterns, weather forecasts, and grid operations. By dynamically managing energy supply and demand, AI-powered smart grids can improve grid stability, increase the integration of renewable energy sources, and enhance overall energy efficiency.
9.2 Energy Storage
Efficient energy storage is crucial for maximizing the utilization of renewable energy sources. AI can help optimize energy storage systems by analyzing energy generation and consumption patterns, weather forecasts, and grid conditions. AI algorithms can predict energy demand and determine the optimal storage capacity and charging/discharging strategies, enhancing the overall efficiency and reliability of renewable energy systems.
9.3 Solar and Wind Power Optimization
AI can improve the efficiency and performance of solar and wind power systems. Machine learning algorithms can optimize the positioning of solar panels and wind turbines, considering factors such as weather conditions, topography, and energy demand. By maximizing energy generation and minimizing downtime, AI helps to increase the share of renewable energy in the overall energy mix and reduce reliance on fossil fuels.
10. Future Prospects of AI and Environmental Impact
10.1 Technological Innovations
Technological advancements in AI have the potential to drive sustainable development and reduce the environmental impact. Continued research and development efforts can lead to more energy-efficient algorithms, hardware, and infrastructure. By incorporating environmental considerations into AI design and development, future innovations may help address environmental challenges and enable a more sustainable and greener AI ecosystem.
10.2 Collaborative Efforts
Addressing the environmental impact of AI requires collaborative efforts from various stakeholders, including governments, industries, researchers, and environmental organizations. By working together, these stakeholders can develop and implement policies, standards, and practices that promote sustainable AI deployment and mitigate the negative environmental consequences associated with AI technologies.
10.3 Ethical Considerations
As AI continues to evolve and play a significant role in environmental management, ethical considerations become crucial. Transparency, fairness, and inclusivity should be integral to AI decision-making processes and ensure that the benefits and risks of AI are distributed equitably. By prioritizing ethical considerations, stakeholders can establish a framework that ensures the responsible and sustainable utilization of AI in addressing environmental challenges.