The AI Industry Trends To Watch
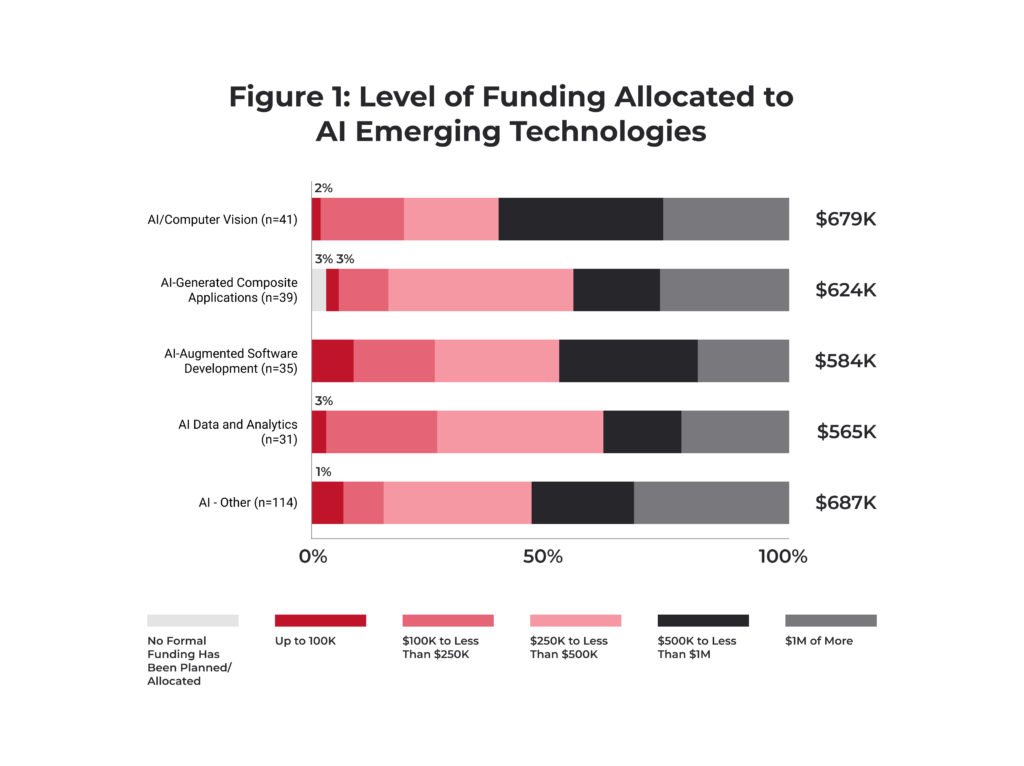
AI, short for Artificial Intelligence, is revolutionizing the world as we know it. From virtual assistants like Siri to self-driving cars, AI technology is becoming increasingly prevalent in our daily lives. But what are the latest AI industry trends that we should be keeping an eye on? In this article, we will explore some of the most exciting developments in AI and how they are shaping the future. So grab your cup of coffee and get ready to embark on a journey into the fascinating world of AI industry trends.
1. The Advancement of Natural Language Processing (NLP)
1.1. Improvements in Voice Assistants
Voice assistants, such as Amazon’s Alexa, Apple’s Siri, and Google Assistant, have become increasingly prevalent in our daily lives. The advancements in Natural Language Processing (NLP) have played a crucial role in enhancing the capabilities of these voice assistants. NLP allows voice assistants to understand and interpret human language, enabling users to interact with them through voice commands.
Over the years, voice assistants have significantly improved in their accuracy and comprehension of spoken language. They can now understand contextual cues, handle complex commands, and provide more accurate responses. This progress has made voice assistants more user-friendly and efficient, enabling users to perform tasks such as setting reminders, checking the weather, playing music, and even controlling smart home devices with ease.
1.2. Language Translation Services
Language translation services have also witnessed remarkable advancements due to NLP. These services, like Google Translate and Microsoft Translator, rely on sophisticated machine learning algorithms to translate text from one language to another.
With NLP, language translation services have become more accurate and natural-sounding. They can now handle complex sentences, idioms, and even dialects, making it easier for people all around the world to communicate and access information in their native languages. This progress has been particularly beneficial for travelers, businesses operating globally, and individuals seeking to bridge language barriers.
1.3. Increased Adoption in Customer Service
The application of NLP in customer service has transformed the way businesses interact with their customers. Chatbots powered by NLP algorithms are increasingly being used to provide automated customer support and assist in resolving customer queries.
By leveraging NLP, businesses can offer round-the-clock support, reduce customer service costs, and provide quick and accurate responses to customer inquiries. Chatbots can understand natural language inputs, interpret customer intents, and provide relevant information or route the customer to the appropriate department for further assistance. This adoption of NLP in customer service has improved customer satisfaction and streamlined the overall customer experience.
1.4. Enhanced Text Analytics
NLP has greatly improved text analytics capabilities, enabling organizations to extract valuable insights and information from large volumes of unstructured text data. With the increasing availability of online content and social media interactions, text analytics has become crucial in understanding customer sentiments, conducting market research, and detecting emerging trends.
Through NLP techniques, organizations can analyze text data to identify patterns, sentiments, and key themes. This helps them make data-driven decisions, improve business strategies, and enhance customer engagement. Text analytics powered by NLP has also assisted in automating processes such as content categorization, document summarization, and sentiment analysis, saving time and resources for businesses across various industries.
2. The Rise of Machine Learning
2.1. Deep Learning
Deep Learning, a subset of Machine Learning, has gained significant traction in recent years. It involves training artificial neural networks with large amounts of data to enable them to learn and make predictions or decisions without explicitly programmed instructions.
Deep Learning has revolutionized various AI applications, including computer vision, natural language processing, and speech recognition. It has led to breakthroughs in tasks such as image classification, object recognition, and speech synthesis. The ability of deep learning models to learn from vast amounts of data and automatically extract complex features has made them highly effective in solving real-world problems.
2.2. AutoML
AutoML, short for Automated Machine Learning, addresses the challenges faced by organizations in deploying Machine Learning models. It automates various steps of the machine learning workflow, including data preprocessing, feature engineering, model selection, and hyperparameter tuning.
By leveraging AutoML tools and platforms, organizations without extensive expertise in Machine Learning can build and deploy models efficiently. AutoML reduces the barrier to entry for businesses, making it easier and more accessible to implement AI solutions and derive insights from data. This advancement in Machine Learning democratizes AI, allowing organizations of all sizes and industries to benefit from its applications.
2.3. Federated Learning
Federated Learning is an emerging approach in Machine Learning that enables training models on decentralized data without sharing the sensitive raw data. It addresses privacy concerns associated with centralized data collection and allows models to be trained on data distributed across multiple devices or servers.
In Federated Learning, the training process occurs on the edge devices, such as smartphones or IoT devices, without transferring raw data to a central server. Only model updates or aggregated information is shared, ensuring data privacy and security. Federated Learning has promising applications in healthcare, where sensitive patient data can be utilized for model training without compromising privacy regulations.
3. Ethical Considerations in AI
3.1. Bias in AI Algorithms
As AI becomes increasingly integrated into various aspects of society, concerns surrounding bias in AI algorithms have come to the forefront. AI algorithms are trained on diverse datasets, which might unintentionally contain biases present in the data or underlying societal biases.
To address this issue, there is a growing need for AI practitioners to focus on improving algorithmic fairness and eliminating biases. This can be achieved through careful dataset curation, algorithm design, and ongoing monitoring and evaluation of AI systems. It is crucial to ensure that AI algorithms are transparent, accountable, and deliver fair treatment to all individuals, regardless of race, gender, or any other protected characteristic.
3.2. Privacy Concerns
The proliferation of AI technologies that collect and process vast amounts of personal data has raised legitimate concerns about privacy. AI systems often rely on collecting and analyzing user data to improve their performance and deliver personalized experiences.
To maintain user trust and protect privacy, organizations need to prioritize ethical data practices. Implementing robust privacy policies, obtaining informed consent, and adopting privacy-enhancing technologies such as differential privacy can help address these concerns. It is essential to strike the right balance between leveraging user data to enhance AI capabilities and respecting individual privacy rights.
3.3. Transparency and Accountability
AI systems often employ complex algorithms and decision-making processes that might not be readily understandable to end-users or even the developers themselves. This lack of transparency raises concerns about the accountability of AI systems.
To ensure transparency and accountability, organizations should strive to develop AI systems that are explainable, auditable, and subject to external scrutiny. Open-source AI frameworks, explainable AI techniques, and third-party audits can help mitigate the opacity of AI decision-making and promote trust and accountability.
3.4. Fairness and Equal Treatment
Promoting fairness and equal treatment is a critical ethical consideration in AI. AI systems should not perpetuate or amplify existing societal biases or discrimination. Instead, they should strive to provide equitable outcomes for all individuals.
To achieve fairness, organizations should prioritize diversity and inclusion in AI development teams to ensure the systems are built with a broad range of perspectives. Regular audits and evaluations should also be conducted to identify and address any biases or disparate impacts. The development and implementation of AI ethics guidelines and frameworks can further promote fairness and avoid discriminatory outcomes.
4. AI in Healthcare
4.1. Medical Imaging and Diagnostics
AI has made significant advancements in the field of medical imaging and diagnostics. AI-powered algorithms can analyze medical images, such as X-rays, CT scans, and MRIs, to aid in the detection and diagnosis of various diseases and conditions.
By leveraging deep learning techniques, AI algorithms can quickly and accurately identify anomalies, assist radiologists in interpreting images, and improve diagnostic accuracy. This advancement in medical imaging and diagnostics has the potential to enhance patient outcomes, reduce healthcare costs, and enable earlier detection of diseases.
4.2. Drug Discovery and Development
AI is also transforming the drug discovery and development process. Traditional drug discovery can be time-consuming and costly, with a high failure rate. AI algorithms can expedite and improve this process by analyzing large volumes of data, identifying patterns, and predicting the effectiveness of potential drug candidates.
AI-driven drug discovery allows researchers to explore a broader range of molecules and assess their potential efficacy and safety. By leveraging AI tools, pharmaceutical companies can accelerate the development of new drugs, bring them to market faster, and potentially address unmet medical needs more efficiently.
4.3. Patient Monitoring and Personalized Medicine
AI technologies are revolutionizing patient monitoring and enabling personalized medicine. By analyzing patient data, such as electronic health records, wearable sensor data, and genetic information, AI algorithms can provide real-time insights and personalized recommendations for healthcare interventions.
AI-powered predictive analytics can help identify individuals at high risk of developing certain conditions, enabling proactive interventions and personalized treatment plans. This not only improves patient outcomes but also reduces healthcare costs by avoiding unnecessary treatments or hospitalizations. AI-driven patient monitoring also allows for remote monitoring, enabling healthcare providers to monitor patients’ health conditions from a distance and intervene when necessary.
5. Autonomous Vehicles and Transportation
5.1. Self-Driving Cars
One of the most widely recognized applications of AI is in the development of self-driving cars. Autonomous vehicles rely on AI algorithms and sensors to perceive the environment, make decisions, and navigate safely without human intervention.
The advancements in AI, particularly in computer vision, machine learning, and sensor technologies, have paved the way for significant progress in autonomous vehicle development. Self-driving cars have the potential to revolutionize transportation by reducing accidents, improving traffic efficiency, and enhancing mobility for individuals who are unable to drive themselves. However, challenges such as regulatory frameworks, safety concerns, and public acceptance need to be addressed for successful widespread adoption.
5.2. Intelligent Traffic Management Systems
AI-based intelligent traffic management systems are transforming the way we manage and optimize traffic flow in cities. These systems leverage real-time data from various sources, including sensors, cameras, and GPS devices, to monitor traffic conditions and make intelligent decisions to optimize traffic flow.
By analyzing and predicting traffic patterns, AI-driven traffic management systems can dynamically adjust traffic signals, suggest alternative routes, and optimize traffic flow to reduce congestion and travel times. This leads to improved road safety, reduced fuel consumption, and enhanced overall transportation efficiency.
5.3. Predictive Maintenance in Transportation
AI is also playing a crucial role in predictive maintenance in transportation. By analyzing sensor data from vehicles, AI algorithms can detect anomalies, predict failures, and recommend proactive maintenance actions.
Predictive maintenance enables transportation companies to identify potential issues before they lead to breakdowns or accidents, minimizing downtime and optimizing maintenance schedules. This proactive approach helps reduce equipment repair costs, improve safety, and ensure the reliability and efficiency of the transportation infrastructure.
6. AI in Financial Services
6.1. Fraud Detection and Prevention
AI is revolutionizing fraud detection and prevention in the financial services industry. Machine learning algorithms can analyze vast amounts of data, including transaction patterns, customer behavior, and historical fraud cases, to identify suspicious activities and detect anomalies.
By leveraging AI, financial institutions can enhance their fraud detection capabilities, reduce false positives, and mitigate risks. AI-powered systems can identify emerging fraud patterns, adapt to evolving fraud techniques, and provide real-time alerts to prevent fraudulent transactions. This helps protect both financial institutions and their customers from financial losses and maintain trust in the financial system.
6.2. Algorithmic Trading
AI algorithms are extensively used in algorithmic trading to automate the process of buying and selling financial instruments. By analyzing real-time market data, news feeds, and historical trading patterns, AI algorithms can make rapid and data-driven trading decisions.
Algorithmic trading powered by AI offers several advantages, including faster trade execution, reduced transaction costs, and increased liquidity. These systems can quickly analyze vast amounts of data, identify trading opportunities, and execute trades at optimal prices. However, careful risk management and monitoring are necessary to ensure the stability and integrity of algorithmic trading systems.
6.3. Personalized Banking
AI is transforming the way banks interact with their customers through personalized banking services. By analyzing customer data, AI-powered systems can understand individual preferences, predict financial needs, and provide personalized recommendations.
Personalized banking enables banks to offer tailored financial products, such as investment advice, loan recommendations, and insurance plans, based on customer profiles and goals. AI algorithms can also automate customer interactions, such as chatbots providing personalized support or virtual assistants managing personal finances. This improves customer engagement, enhances the customer experience, and strengthens the relationship between banks and their customers.
7. AI in Manufacturing and Robotics
7.1. Industrial Automation
AI-enabled industrial automation is revolutionizing manufacturing processes by leveraging robotics, machine learning, and computer vision technologies. AI-powered robots can perform repetitive and physically demanding tasks with precision, speed, and efficiency.
By automating manufacturing processes, AI systems can significantly improve productivity, reduce errors, and optimize resource utilization. Robots equipped with AI algorithms can adapt to changing production needs, handle variations in product designs, and collaborate with human workers on complex tasks. This not only enhances manufacturing efficiency but also improves workplace safety and frees up human workers to focus on more creative and complex roles.
7.2. Quality Control and Inspections
AI is enhancing quality control and inspection processes in manufacturing industries. By analyzing product images or sensor data, AI algorithms can detect defects, deviations, or anomalies that might be difficult for human inspectors to identify.
AI-driven quality control systems can identify defects at a faster pace and with higher accuracy, improving product quality and reducing production costs. These systems can also learn from previous inspection data to continuously improve their defect detection capabilities and provide real-time feedback to production processes, ensuring the highest standards of product quality.
7.3. Collaborative Robots (Cobots)
Collaborative robots, or cobots, are AI-powered robots designed to work collaboratively with human workers in industrial settings. Cobots can perform tasks that require human dexterity, precision, or decision-making in collaboration with their human counterparts.
Cobots excel in scenarios where humans and robots can combine their strengths, such as handling delicate materials, performing repetitive tasks, or assisting with complex assembly processes. By leveraging AI algorithms, cobots can adapt to human behavior, understand instructions, and work safely alongside humans without the need for dedicated safety barriers. This enables increased productivity, improved worker satisfaction, and enhanced workplace safety.
8. AI and Cybersecurity
8.1. Threat Detection and Mitigation
AI is playing a crucial role in enhancing cybersecurity by providing advanced threat detection and mitigation capabilities. AI algorithms can analyze massive amounts of data, including network traffic, user behavior, and system log data, to identify patterns and detect anomalies that might indicate cyber threats.
By leveraging AI-powered threat detection systems, organizations can quickly identify and respond to security incidents, minimizing the potential impact of cyber attacks. These systems can detect known and unknown threats, classify them based on their severity, and provide real-time alerts or automated responses to mitigate the risks. AI-driven threat detection enhances cybersecurity defenses, reducing the time and resources required to detect and respond to evolving cyber threats.
8.2. User Authentication and Credentialing
AI technologies are improving user authentication and credentialing processes, making them more secure and convenient. Biometric authentication, such as facial recognition or fingerprint scanning, leverages AI algorithms to verify users’ identities accurately and efficiently.
AI-powered authentication systems can analyze unique biometric features, compare them with stored templates, and grant or deny access based on the match. This strengthens cybersecurity defenses by reducing the reliance on passwords, which can be easily compromised. AI-driven authentication also provides a seamless user experience, eliminating the need for manual input of credentials while maintaining high levels of security.
8.3. AI-Enabled Security Analytics
AI-enabled security analytics is transforming the way organizations monitor and analyze security-related data. By analyzing diverse data sources, including log files, user behavior, and threat intelligence feeds, AI algorithms can detect suspicious activities, correlate events, and identify potential security incidents.
AI-powered security analytics enables organizations to proactively identify security vulnerabilities, anticipate threats, and respond quickly to potential breaches. These systems can identify patterns indicating malicious activities, prioritize alerts based on their relevance and severity, and assist security analysts in investigating and mitigating threats. AI-driven security analytics enhances the efficiency and effectiveness of cybersecurity operations, enabling organizations to stay one step ahead of sophisticated cyber attackers.
9. AI in Agriculture
9.1. Crop Monitoring and Optimization
AI is revolutionizing agriculture by enabling efficient crop monitoring and optimization. By analyzing satellite imagery, weather data, and sensor data from farms, AI algorithms can provide valuable insights and recommendations to farmers.
AI-powered systems can assess crop health, identify areas of pest infestation or nutrient deficiency, and optimize irrigation and fertilization practices. This allows farmers to optimize resource utilization, minimize environmental impact, and improve crop yields. By monitoring crop growth and health in real-time, AI-driven agriculture enables early intervention, reducing crop losses and ensuring sustainable agricultural practices.
9.2. Livestock Monitoring and Management
AI technologies are enhancing livestock monitoring and management practices. By leveraging sensors, image recognition, and machine learning algorithms, AI systems can monitor the health, behavior, and productivity of livestock.
AI-powered livestock monitoring enables early detection of health issues, such as diseases or injuries, ensuring timely intervention and minimizing the spread of infectious diseases. These systems can also analyze data on feed consumption, weight gain, and other parameters to optimize nutrition and breeding strategies and improve overall productivity. AI-driven livestock management promotes animal welfare, reduces veterinary costs, and enhances the efficiency of livestock farming.
9.3. Precision Agriculture
Precision agriculture, enabled by AI, is transforming agricultural practices by providing targeted and site-specific interventions. By integrating data from various sources, such as soil sensors, weather data, and historical crop performance, AI algorithms can optimize agricultural practices, from planting and fertilizing to harvesting.
AI-driven precision agriculture enables farmers to tailor farming practices to specific areas, optimizing resource allocation, minimizing environmental impact, and maximizing crop productivity. These systems can generate customized recommendations on the ideal planting time, fertilizer and pesticide application rates, and irrigation schedules. Precision agriculture not only enhances agricultural efficiency but also promotes sustainable farming practices and minimizes the use of inputs, such as water and chemicals.
10. Integration of AI and Internet of Things (IoT)
10.1. Smart Home Automation
The integration of AI and IoT has paved the way for smart home automation. AI algorithms can analyze data from various smart devices, such as thermostats, security cameras, and voice assistants, to understand user preferences and automate home management tasks.
AI-powered smart home systems can learn user behavior patterns, adjust temperature settings, schedule appliances, and optimize energy consumption based on user preferences and real-time data. This improves convenience, energy efficiency, and overall home management experience. Smart home automation also enhances home security by allowing AI systems to detect and respond to potential threats, such as unauthorized access or abnormal activities.
10.2. Industrial IoT Applications
The combination of AI and IoT technologies is revolutionizing industrial applications. By connecting industrial equipment, sensors, and data analytics platforms, AI-powered industrial IoT systems can optimize manufacturing processes, reduce downtime, and improve overall productivity.
AI algorithms analyze real-time data from IoT devices to detect anomalies, identify performance bottlenecks, and predict equipment failures. By proactively addressing maintenance needs and optimizing production schedules, AI-enabled industrial IoT enhances efficiency, reduces costs, and ensures the seamless operation of industrial facilities. The integration of AI and IoT also enables real-time monitoring and control of critical infrastructure, ensuring safety and reliability.
10.3. AI-Driven Predictive Maintenance
The integration of AI and IoT technologies enables AI-driven predictive maintenance. By collecting and analyzing real-time sensor data from various machines and equipment, AI algorithms can predict maintenance needs, identify potential failures, and recommend proactive maintenance actions.
Predictive maintenance powered by AI and IoT helps eliminate unplanned downtime, save costs associated with reactive maintenance, and optimize maintenance schedules. By identifying and addressing maintenance needs in advance, AI-driven predictive maintenance enhances equipment reliability, improves operational efficiency, and extends the lifespan of assets. Organizations can avoid costly disruptions, optimize resource utilization, and ensure the continuous availability of critical infrastructure.
In conclusion, the advancements in AI technologies have catalyzed transformations across various industries. The improvement in Natural Language Processing has revolutionized voice assistants, language translation services, and customer service. Machine Learning, particularly Deep Learning, AutoML, and Federated Learning, has propelled advancements in various AI applications. Ethical considerations are playing an increasingly crucial role in the development and deployment of AI, addressing issues such as bias, privacy, transparency, and fairness. AI’s impact in healthcare, autonomous vehicles, financial services, manufacturing, cybersecurity, agriculture, and the integration with IoT is driving innovation, improving efficiency, and transforming industries. As the AI industry continues to evolve, it is vital to navigate these trends responsibly, ensuring the ethical and responsible adoption of AI to maximize its benefits for society as a whole.