AI In Manufacturing
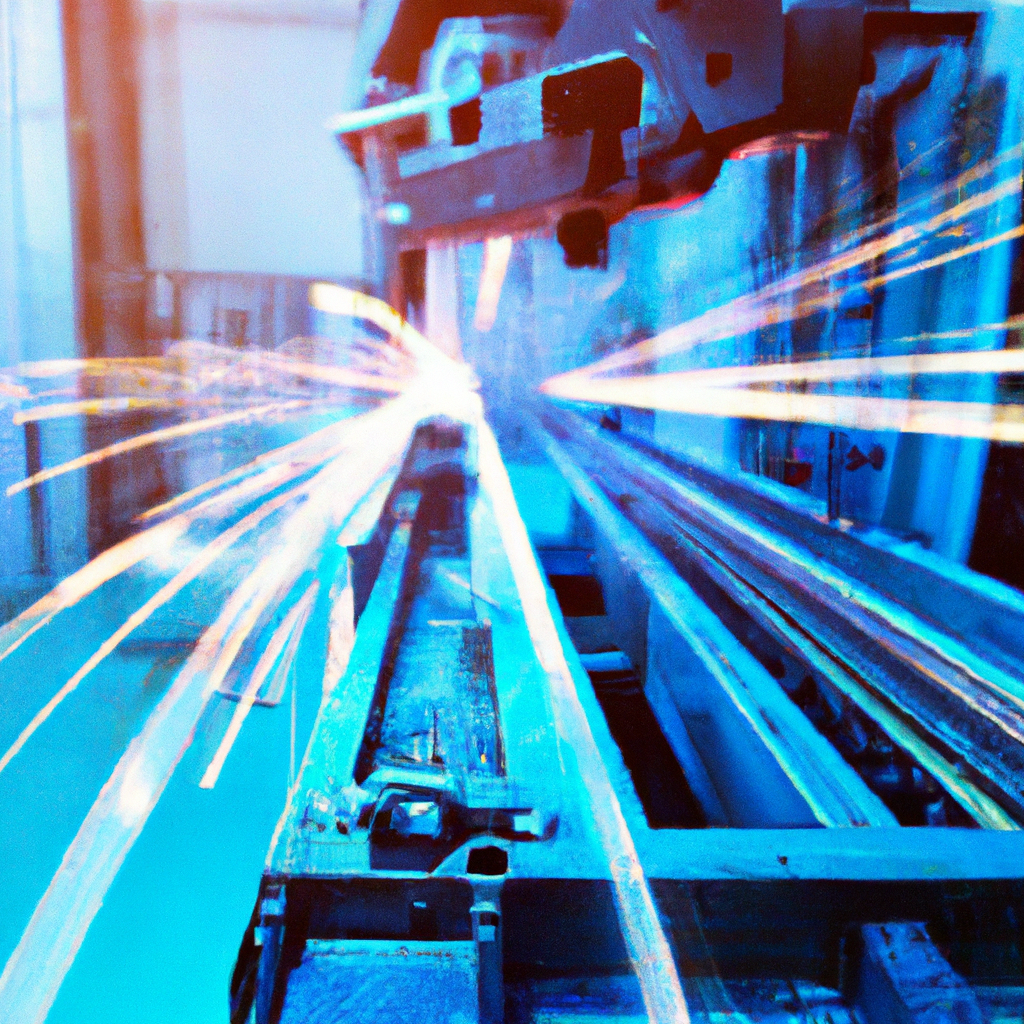
Imagine a world where machines can independently identify and rectify manufacturing defects, optimize production schedules, and improve overall efficiency—all with little to no human intervention. This futuristic vision is becoming an exciting reality with the advent of Artificial Intelligence (AI) in manufacturing. Harnessing the power of machine learning and data analytics, AI is revolutionizing the manufacturing industry, unlocking immense potential for increased productivity, cost savings, and enhanced quality. From predictive maintenance to intelligent supply chain management, this article explores the transformative impact of AI in manufacturing and the boundless opportunities it brings.
Benefits of AI in Manufacturing
Quality Improvement
AI technology plays a crucial role in improving the quality of products in the manufacturing industry. With its advanced algorithms and machine learning capabilities, AI systems can analyze vast amounts of data to detect defects and anomalies in products. By automating the inspection process, AI can identify even the smallest imperfections that may be difficult to spot with the naked eye, ensuring that only high-quality products reach the market. This not only enhances customer satisfaction but also helps manufacturers maintain their reputation for producing top-notch goods.
Increased Efficiency and Productivity
One of the major advantages of integrating AI into the manufacturing process is the significant increase in operational efficiency and productivity. AI-powered automation systems, such as robotic process automation, can carry out repetitive and labor-intensive tasks with great precision and speed. This allows human workers to focus on more complex and value-added activities, ultimately leading to a boost in overall productivity. Moreover, AI algorithms can optimize production schedules, reduce downtime, and minimize waste, further enhancing efficiency in the manufacturing environment.
Predictive Maintenance
AI has revolutionized the concept of maintenance in the manufacturing industry by introducing predictive maintenance techniques. Traditional maintenance practices are often reactive, meaning that equipment is repaired or replaced only after it has failed. This approach can be costly and disruptive to the production process. With AI, manufacturers can implement condition monitoring systems that continuously monitor the health of machinery and equipment in real-time. By analyzing data from sensors and other sources, AI algorithms can detect early signs of potential failures and predict when maintenance is required. This preventive approach not only reduces downtime but also extends the lifespan of equipment, saving costs in the long run.
Cost Reduction
Implementing AI technology in manufacturing can lead to significant cost reductions for companies. By automating various processes, manufacturers can reduce the need for manual labor, resulting in lower labor costs. Additionally, AI-powered production planning systems can optimize resource allocation, minimize waste, and streamline operations, ultimately reducing overall production costs. Moreover, predictive maintenance techniques can help prevent costly equipment breakdowns and minimize unplanned downtime, further reducing expenses. With AI, manufacturers can achieve greater cost efficiency and competitiveness in a highly competitive market.
Improved Safety
Safety is a critical aspect of manufacturing, and AI can play a vital role in enhancing workplace safety. AI-powered systems can monitor real-time safety conditions and identify potential hazards, enabling proactive measures to mitigate risks. For example, computer vision technology can detect unsafe behaviors or conditions and alert workers or supervisors before accidents occur. Additionally, AI algorithms can analyze historical safety data to identify patterns and trends, enabling manufacturers to implement preventive measures to avoid accidents and injuries. By prioritizing safety through AI applications, manufacturers can create a safer working environment for their employees and minimize the risk of workplace accidents.
Challenges of Implementing AI in Manufacturing
High Initial Investment
One of the key challenges in implementing AI in manufacturing is the high initial investment required. Developing and deploying AI systems can be costly, as it involves purchasing advanced technologies, integrating them into existing infrastructures, and training the workforce to operate and maintain the systems. However, it is important to consider that the benefits of AI, such as improved efficiency and productivity, often outweigh the initial costs. Additionally, as AI technology continues to advance and become more accessible, the initial investment may become more affordable for manufacturers of all sizes.
Data Integration and Management
Successful implementation of AI in manufacturing relies heavily on the availability and quality of data. AI systems require large amounts of data to train their algorithms and make accurate predictions or decisions. However, integrating data from various sources within a manufacturing environment can be challenging. Manufacturers need to ensure that data is collected, structured, and stored in a manner that is compatible with AI algorithms. This may require investing in data management systems and processes that can handle the volume, variety, and velocity of data generated in a manufacturing environment.
Skill Gap
Another challenge in implementing AI in manufacturing is the skill gap that exists within the workforce. AI technology is relatively new, and many manufacturing employees may lack the necessary skills and knowledge to effectively operate and leverage AI systems. To overcome this challenge, manufacturers need to invest in training programs and upskilling initiatives to ensure that their workforce is equipped with the skills to work alongside AI technologies. Collaborations with educational institutions and partnerships with AI technology providers can also help bridge the skill gap and ensure a smooth transition to an AI-driven manufacturing environment.
Ethical and Security Concerns
Integrating AI into manufacturing processes raises ethical and security concerns that need to be addressed. For example, AI algorithms may make decisions that have ethical implications, and manufacturers need to establish clear guidelines and rules to ensure that AI systems operate ethically and responsibly. Additionally, the reliance on AI systems introduces potential vulnerabilities in terms of data privacy and security. Manufacturers must implement robust cybersecurity measures to protect sensitive data from unauthorized access or breaches. Collaborating with experts in AI ethics and cybersecurity can help address these concerns and ensure that AI is deployed in a responsible and secure manner.
Resistance to Change
Implementing AI in manufacturing may face resistance from employees who fear that AI will replace their jobs. It is important for manufacturers to communicate the benefits of AI to their workforce and highlight the potential for job enhancement rather than job elimination. By involving employees in the implementation process and providing training and support, manufacturers can help alleviate concerns and create a culture that embraces AI as a tool to improve productivity and job satisfaction. Open and transparent communication is key to overcoming resistance and fostering a positive attitude towards AI implementation.
AI-powered Automation
Robotic Process Automation
Robotic Process Automation (RPA) involves the use of software robots or “bots” to automate repetitive and rule-based tasks in manufacturing. These bots can emulate human actions, such as data entry, data manipulation, and communication, allowing manufacturers to streamline processes, reduce errors, and increase efficiency. RPA can be integrated with existing systems and does not require significant changes to the underlying infrastructure. Manufacturers can significantly benefit from RPA by reducing labor costs, increasing accuracy, and improving overall operational efficiency.
Machine Learning Algorithms
Machine learning algorithms are at the core of AI-powered automation in manufacturing. These algorithms can analyze large amounts of data, identify patterns, and make predictions or decisions without explicit programming. In the manufacturing context, machine learning algorithms can be used for various purposes, such as demand forecasting, quality control, predictive maintenance, and resource optimization. By continuously learning from data, these algorithms can improve their performance over time and help manufacturers make more informed and accurate decisions.
Computer Vision
Computer vision technology enables AI systems to interpret and analyze visual data, such as images and videos. In manufacturing, computer vision can be used for tasks such as quality control, defect detection, and real-time monitoring. For example, AI-powered cameras can inspect products for defects, ensuring that only high-quality items are shipped to customers. Computer vision algorithms can also detect safety hazards and identify unsafe behaviors in the manufacturing environment. By leveraging computer vision technology, manufacturers can improve product quality, enhance safety, and optimize operations.
Natural Language Processing
Natural Language Processing (NLP) enables AI systems to understand and interpret human language. In the manufacturing context, NLP can be used for tasks such as voice recognition, text analysis, and intelligent assistants. For example, manufacturers can use NLP-powered intelligent assistants to provide real-time instructions and guidance to workers on the shop floor. NLP algorithms can also analyze customer feedback and reviews, helping manufacturers gain valuable insights and improve their products or services. By leveraging NLP, manufacturers can enhance communication, streamline processes, and improve overall efficiency.
AI in Quality Control and Inspection
Automated Defect Detection
AI systems can automate the process of defect detection in manufacturing, ensuring that only high-quality products are released to the market. By analyzing data from sensors, cameras, and other sources, AI algorithms can identify defects, such as scratches, dents, or color variations, that may be difficult to spot with the naked eye. Automated defect detection not only improves product quality but also reduces the need for manual inspection, saving time and costs for manufacturers.
Real-time Monitoring
Real-time monitoring is a critical aspect of quality control in manufacturing, and AI can significantly enhance this process. AI systems can continuously monitor various parameters, such as temperature, pressure, or vibration, in real-time. By analyzing data from sensors and other sources, AI algorithms can detect deviations from normal operating conditions and trigger alerts or preventive actions. Real-time monitoring helps manufacturers identify potential issues early on and take immediate corrective measures, minimizing the risk of defects or failures.
Statistical Analysis
Statistical analysis is an integral part of quality control in manufacturing, and AI can play a crucial role in this area. AI algorithms can analyze large amounts of data to identify trends, patterns, and correlations that may be challenging for humans to perceive. By leveraging statistical analysis, manufacturers can gain insights into the root causes of defects or quality issues, enabling them to implement targeted improvements. Statistical analysis also helps manufacturers track key performance indicators and continuously monitor quality metrics, ensuring that products meet the required standards.
Fault Prediction and Prevention
AI-powered predictive analytics can help manufacturers predict and prevent faults or failures in the production process. By analyzing historical data, AI algorithms can identify patterns or anomalies that may indicate an impending fault. Manufacturers can then take proactive measures, such as adjusting process parameters or scheduling maintenance, to prevent the fault from occurring. Fault prediction and prevention not only minimize downtime but also reduce the risk of defective products reaching the market, leading to increased customer satisfaction.
Predictive Maintenance with AI
Condition Monitoring
Condition monitoring is a key component of predictive maintenance in manufacturing, and AI can greatly enhance this process. AI systems can continuously monitor the health and performance of equipment by analyzing data from sensors, meters, and other sources. By detecting early signs of deterioration or abnormalities, AI algorithms can provide manufacturers with insights into the condition of their assets. This enables manufacturers to pinpoint potential issues and schedule maintenance activities before major failures or breakdowns occur.
Anomaly Detection
Anomaly detection is a crucial capability of AI systems in predictive maintenance. AI algorithms can analyze large amounts of sensor data to identify patterns or behaviors that deviate from the norm. By detecting anomalies, such as unusual vibrations or temperature fluctuations, AI systems can alert manufacturers to potential faults or failures. Anomaly detection not only helps prevent costly breakdowns but also minimizes disruptions to the production process, ensuring smooth operations.
Failure Prediction
By analyzing historical data and identifying patterns, AI algorithms can predict equipment failures before they happen. This allows manufacturers to take proactive measures, such as replacing components or scheduling maintenance, to prevent failures and minimize downtime. Failure prediction based on AI-driven analytics helps manufacturers optimize their maintenance strategies, reduce costs, and extend the lifespan of their equipment.
Optimized Maintenance Scheduling
AI can optimize maintenance scheduling by taking into account various factors, such as equipment condition, production requirements, and resource availability. By analyzing data and considering multiple variables, AI algorithms can determine the most efficient and cost-effective maintenance schedule. Optimized maintenance scheduling reduces downtime, minimizes disruptions to the production process, and ensures that maintenance activities are carried out at the right time and with the right resources.
AI in Supply Chain Management
Demand Forecasting and Planning
AI technology can significantly improve demand forecasting and planning in the manufacturing industry. By analyzing historical sales data, market trends, and other relevant factors, AI algorithms can make accurate predictions about future demand. This enables manufacturers to optimize their production schedules, align their inventory levels with expected demand, and avoid overstocking or stockouts. With AI-powered demand forecasting and planning, manufacturers can enhance customer satisfaction, minimize costs, and improve overall supply chain efficiency.
Inventory Management
Efficient inventory management is critical for manufacturing companies to meet customer demand while minimizing costs. AI systems can analyze various factors, such as sales data, production schedules, and supplier information, to optimize inventory levels. By using AI algorithms to determine reorder points, safety stock levels, and replenishment strategies, manufacturers can ensure that the right amount of inventory is available at the right time. AI-powered inventory management reduces the risk of stockouts, minimizes inventory holding costs, and improves cash flow.
Logistics Optimization
AI can play a pivotal role in optimizing logistics operations in the manufacturing industry. By analyzing data on transportation routes, delivery schedules, and carrier performance, AI algorithms can optimize the selection and coordination of logistics providers. This helps manufacturers reduce transportation costs, improve delivery speed, and enhance customer satisfaction. AI-powered logistics optimization also enables manufacturers to respond quickly to changes in demand or supply, ensuring efficient and agile supply chain operations.
Route Optimization
AI technology can optimize route planning for manufacturers, reducing transportation costs and improving delivery efficiency. By considering factors such as distance, traffic conditions, delivery windows, and vehicle capacities, AI algorithms can determine the most optimal routes for product distribution. Route optimization minimizes fuel consumption, reduces travel time, and enhances the overall sustainability of the supply chain. Manufacturers can benefit from cost savings and improved customer service by leveraging AI-powered route optimization.
Improving Operational Efficiency with AI
Production Planning and Scheduling
AI technology can revolutionize production planning and scheduling in the manufacturing industry. By analyzing various factors, such as customer demand, resource availability, and production capacities, AI algorithms can generate optimized production plans and schedules. This helps manufacturers minimize changeovers, reduce idle time, and improve resource utilization. AI-powered production planning and scheduling ensure that production activities are aligned with demand, leading to increased efficiency and on-time delivery.
Resource Allocation
Efficient resource allocation is crucial for manufacturers to optimize their operations and maximize productivity. AI systems can analyze data on resource availability, production requirements, and other relevant factors to allocate resources effectively. By optimizing resource allocation, manufacturers can minimize bottlenecks, reduce idle time, and ensure that resources are utilized efficiently. AI-powered resource allocation leads to improved operational efficiency, increased productivity, and reduced costs.
Workforce Optimization
AI technology can help manufacturers optimize their workforce by analyzing data on productivity, skills, and job requirements. By considering factors such as employee availability, skill levels, and task specifications, AI algorithms can generate optimized work schedules and assignments. This enables manufacturers to allocate the right workforce to the right tasks and maximize productivity. AI-powered workforce optimization also promotes employee satisfaction by ensuring a balanced workload and providing opportunities for skill development.
Energy Management
AI can significantly improve energy management in manufacturing, leading to cost savings and environmental benefits. AI systems can analyze data from energy meters, sensors, and other sources to identify energy-saving opportunities and detect anomalies. By optimizing energy consumption and reducing energy wastage, manufacturers can lower operational costs and minimize their carbon footprint. AI-powered energy management enables manufacturers to achieve sustainability goals while maintaining efficient operations.
AI-based Safety and Risk Management
Real-time Safety Monitoring
AI technology can enable real-time safety monitoring in the manufacturing environment, helping to prevent accidents and ensure a safe working environment for employees. AI-powered systems can analyze data from sensors, cameras, and other sources to identify potential safety hazards or unsafe behaviors. By detecting and alerting workers or supervisors to unsafe conditions in real-time, AI systems can help prevent accidents before they occur. Real-time safety monitoring enhances workplace safety and reduces the risk of injuries or fatalities.
Hazard Identification
AI algorithms can play a vital role in identifying and mitigating hazards in the manufacturing industry. By analyzing data on workplace conditions, equipment performance, and other relevant factors, AI systems can identify potential hazards and provide actionable insights for risk mitigation. Manufacturers can use AI-powered hazard identification to implement preventive measures, such as redesigning processes, improving safety protocols, or providing additional training. Hazard identification based on AI technology helps manufacturers create safer working environments and protect their employees.
Risk Assessment and Mitigation
AI technology can assist manufacturers in assessing and mitigating risks associated with their operations. By analyzing data on various risk factors, such as equipment reliability, supply chain vulnerabilities, or regulatory compliance, AI algorithms can identify potential risks and quantify their impacts. This enables manufacturers to develop risk mitigation strategies and prioritize resources accordingly. AI-based risk assessment and mitigation help manufacturers proactively manage risks, reduce potential losses, and ensure business continuity.
Emergency Response Planning
AI can enhance emergency response planning in the manufacturing industry, helping manufacturers prepare for and respond to critical incidents effectively. AI systems can analyze historical and real-time data on emergency scenarios, such as fires, natural disasters, or equipment failures, to develop robust response plans. By simulating various emergency scenarios and optimizing response strategies, AI algorithms can help manufacturers minimize the impact of emergencies and protect lives and assets. AI-powered emergency response planning improves safety preparedness and ensures a swift and coordinated response in critical situations.
Human-Machine Collaboration
Cobotics
Cobotics, also known as collaborative robotics, involves the collaboration of humans and robots in the manufacturing environment. AI-powered robots can work alongside human workers, complementing their skills and capabilities. While robots handle repetitive or physically demanding tasks, humans can focus on more complex and cognitive activities. Cobotics improves productivity, reduces the risk of injuries, and enhances overall efficiency. Manufacturers benefit from the combination of human expertise and robot precision, resulting in a more productive and harmonized workforce.
Augmented Reality
Augmented Reality (AR) technology enhances human-machine collaboration in manufacturing. By overlaying digital information onto the physical environment, AR enables workers to access real-time instructions, data, or visualizations during their tasks. AI-powered AR systems can guide workers through complex assembly processes, provide contextual information, or assist in quality control inspections. Augmented Reality improves accuracy, reduces errors, and accelerates learning curves for new workers. Manufacturers can leverage AR to optimize their operations, streamline training processes, and enhance worker performance.
Virtual Reality
Virtual Reality (VR) technology can transform human-machine collaboration in manufacturing by creating immersive and interactive experiences. AI-powered VR systems can simulate realistic training scenarios, allowing workers to practice their skills in a virtual environment. VR can also be used for remote collaboration, enabling experts from different locations to collaborate and solve problems together. By leveraging VR, manufacturers can improve the efficiency and effectiveness of training programs, reduce the risk of errors, and enhance knowledge sharing among their workforce.
Intelligent Assistants
Intelligent assistants, also known as digital or virtual assistants, can enhance human-machine collaboration in the manufacturing industry. AI-powered intelligent assistants can understand natural language and provide real-time guidance or support to workers. For example, intelligent assistants can answer questions, provide instructions, or retrieve relevant information from databases. By leveraging intelligent assistants, manufacturers can improve communication, facilitate knowledge transfer, and enhance overall operational efficiency. Intelligent assistants enable workers to access information and expertise on-demand, leading to increased productivity and job satisfaction.
Implications and Future Outlook
Job Displacement and Upskilling
The implementation of AI in manufacturing raises concerns about job displacement and the need for upskilling the workforce. While AI technology may automate certain tasks and roles, it also creates new opportunities for workers to take on more complex and value-added activities. Manufacturers need to prioritize upskilling initiatives to ensure that their employees have the necessary skills to work alongside AI systems. By investing in training programs and promoting a culture of continuous learning, manufacturers can prepare their workforce for the future and maximize the potential benefits of AI technology.
Data Privacy and Security
Integrating AI into manufacturing processes brings about concerns regarding data privacy and security. AI systems rely on large amounts of data, including sensitive information, to train their algorithms and make accurate predictions or decisions. Manufacturers need to implement robust cybersecurity measures to protect data from unauthorized access, breaches, or misuse. Additionally, manufacturers must adhere to data privacy regulations and establish clear guidelines for the ethical use of AI systems. By prioritizing data privacy and security, manufacturers can build trust with their customers and stakeholders while harnessing the power of AI.
Regulatory Frameworks
The rapid advancement of AI in manufacturing raises the need for adequate regulatory frameworks to ensure responsible and ethical use of the technology. Governments and regulatory bodies need to establish guidelines and standards addressing various aspects of AI implementation, such as data privacy, transparency, accountability, and safety. Manufacturers must comply with these regulations and ensure that their AI systems operate within legal and ethical boundaries. Clear regulatory frameworks promote trust and confidence in AI technology and help foster its responsible adoption in the manufacturing industry.
Advancements in AI Technology
The future outlook for AI in manufacturing is promising, as advancements in AI technology continue to push the boundaries of what is possible. A key area of focus is the development of more advanced machine learning algorithms that can handle increasingly complex tasks and analyze vast amounts of data in real-time. Other areas of advancement include natural language processing, computer vision, and robotics, which will further enhance the capabilities of AI in manufacturing. As AI technology evolves, manufacturers can expect even greater benefits in terms of efficiency, productivity, quality, and safety.
In conclusion, AI has emerged as a game-changer in the manufacturing industry, offering a wide range of benefits and opportunities. From quality improvement and increased efficiency to predictive maintenance and cost reduction, AI technology is transforming the way manufacturers operate. By overcoming challenges such as high initial investment, data integration, skill gap, ethical concerns, and resistance to change, manufacturers can harness the power of AI to optimize their operations and gain a competitive edge. With AI-powered automation, quality control and inspection, predictive maintenance, supply chain management, operational efficiency, safety and risk management, human-machine collaboration, as well as its implications and future outlook, the impact of AI in manufacturing is set to revolutionize the industry and drive innovation in the years to come.