The AI Safety Challenges To Overcome
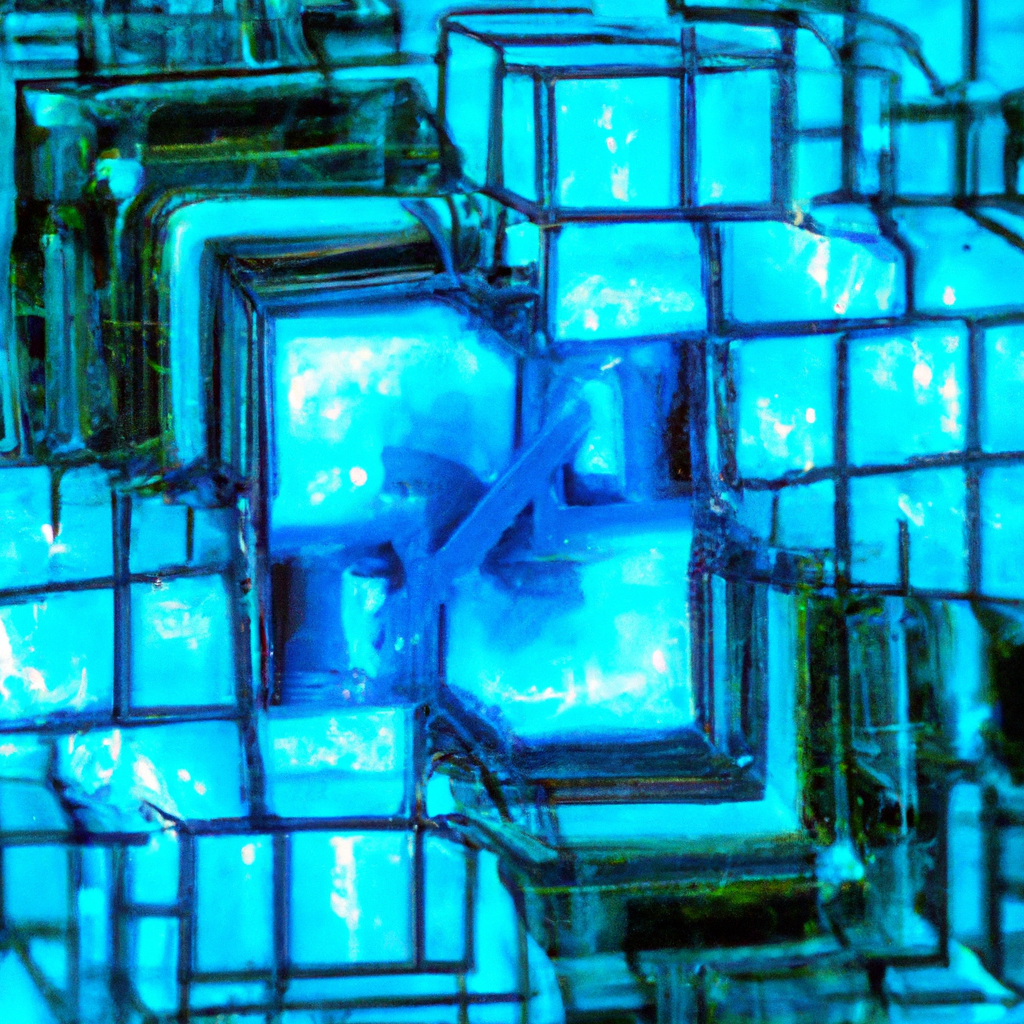
Imagine a world where artificial intelligence (AI) plays an increasingly significant role in our everyday lives, from driving our cars to managing our finances. While this future may seem exciting, there are also important challenges that need to be addressed to ensure the safety and reliability of AI systems. In this article, we will explore some of these challenges, examining the potential risks and discussing the measures necessary to overcome them. So, fasten your seatbelt and get ready to delve into the world of AI safety.
1. Technical Limits
1.1 Computing power
Advancements in computing power are vital for the development and implementation of artificial intelligence (AI) systems. However, there are still technical limits that need to be overcome. Current computing power may not be sufficient to tackle complex AI tasks, such as natural language processing or real-time data analysis. As AI continues to evolve, it is crucial to invest in and push the boundaries of computing power to ensure the efficient and effective functioning of AI systems.
1.2 Data availability
AI systems heavily rely on vast amounts of data to train and improve their performance. However, data availability can be a significant challenge. While there is an abundance of data, not all of it is suitable or accessible for AI training purposes. Additionally, certain domains or industries may have limited data, making it difficult to achieve accurate AI models. Efforts should be made to ensure data availability, quality, and diversity, as these factors play a crucial role in enhancing the capabilities of AI systems.
1.3 Algorithmic biases
AI algorithms are designed to make decisions based on patterns in data. However, these algorithms can be influenced by biases present in the data used to train them. Biases can arise from various sources, including societal prejudices or data limitations. It is essential to address algorithmic biases to prevent unfair or discriminatory outcomes. This can be achieved through rigorous data pre-processing, algorithmic fairness research, and diverse perspectives in AI development teams. By ensuring fairness and reducing biases, AI systems can provide more equitable and inclusive solutions.
2. Ethics and Moral Dilemmas
2.1 Value alignment problem
An important challenge in AI development is the value alignment problem. AI systems need to align their actions with human values and ethical principles to avoid potential harm or undesirable outcomes. Determining how to instill and encode such values into AI systems is a complex task. The challenge lies in understanding and incorporating diverse cultural, moral, and ethical perspectives. Researchers and policymakers should engage in interdisciplinary discussions to find consensus on establishing value alignment frameworks that can guide AI decision-making in a way that reflects human values.
2.2 Unintended consequences
As AI systems become more autonomous and capable, there is a growing concern about unintended consequences. AI systems might exhibit behaviors or outcomes that were not anticipated or desired. Without proper safeguards in place, these unintended consequences could range from minor inconveniences to potentially catastrophic events. It is crucial to anticipate and mitigate risks through robust testing, monitoring, and continuous evaluation of AI systems. Adaptable and self-learning AI systems that can identify and correct unintended consequences can help mitigate these risks.
2.3 Privacy and surveillance concerns
The widespread use of AI technologies raises significant concerns about privacy and surveillance. AI systems often rely on collecting and analyzing personal data to deliver personalized services or make decisions. However, this data collection can infringe upon individuals’ privacy rights. Striking a balance between the benefits of AI and protecting privacy is essential. Robust privacy regulations, data anonymization techniques, and transparency in data usage can help alleviate concerns regarding privacy and surveillance, ensuring that AI respects individuals’ rights and freedoms.
3. Human-AI Interaction
3.1 User comprehension and trust
To fully leverage the potential of AI, it is crucial to establish strong human-AI interaction. Users need to understand the capabilities and limitations of AI systems to make informed decisions. Ensuring AI systems are explainable and intuitive can enhance user comprehension and trust. Clear communication about how AI systems arrive at their decisions or recommendations is essential to build trust and enable users to provide valuable feedback. Additionally, continuous user engagement and feedback loops can help improve the overall user experience and address any concerns or issues that arise.
3.2 Job displacement and unemployment
The rise of AI has raised concerns about job displacement and unemployment. As AI systems automate tasks traditionally performed by humans, there is a potential for job losses or drastic changes in the job market. However, history has shown that technological advancements also create new opportunities and change job roles. To address this challenge, it is crucial to invest in re-skilling and up-skilling programs to equip individuals with the necessary skills to adapt to a changing job landscape. Collaborative efforts between governments, businesses, and educational institutions can help ensure a smooth transition and minimize negative impacts.
3.3 Responsibility and accountability
AI systems are designed to make decisions and take actions autonomously. However, this autonomy raises questions of responsibility and accountability. Who should be held responsible for AI system failures or unintended consequences? Establishing clear lines of responsibility and accountability is crucial for addressing potential legal and ethical issues. Developers, policymakers, and regulators must work together to define frameworks that assign responsibility and liability appropriately. Ethical considerations, transparency, and oversight mechanisms are essential to ensure responsible development, deployment, and use of AI systems.
4. Robustness and Security
4.1 Adversarial attacks
AI systems can be vulnerable to adversarial attacks, where malicious actors manipulate inputs to deceive or exploit AI algorithms. Adversarial attacks can have severe consequences, such as misleading decisions or compromised security systems. Developing robust AI models that are resilient to such attacks is essential. Techniques like adversarial training and robust optimization can help enhance the resilience of AI systems to adversarial attacks. Collaborative efforts between researchers, developers, and security experts are crucial to stay ahead of adversarial threats and ensure the security and integrity of AI systems.
4.2 System vulnerabilities
AI systems, like any other software, can have vulnerabilities that can be exploited by malicious actors. Identifying and addressing these vulnerabilities is essential to prevent unauthorized access, data breaches, or other potential harms. Implementing rigorous security measures, such as secure coding practices, regular security audits, and encryption, can help mitigate system vulnerabilities. Ethical hacking and responsible disclosure practices can also play a crucial role in identifying and remedying vulnerabilities before they can be exploited. Continuous monitoring and updates are necessary to ensure the long-term security of AI systems.
4.3 Autonomous weapons proliferation
The development of autonomous weapons powered by AI raises significant ethical concerns. Autonomous weapons have the potential to act independently, raising questions about human oversight and accountability. There is a need for robust regulations and international agreements to prevent the proliferation of autonomous weapons and ensure that human control is maintained. Ethical considerations, transparency, and adherence to international humanitarian law should guide discussions and policies to prevent the misuse of AI technologies for military purposes.
5. Regulation and Governance
5.1 Lack of legal frameworks
The rapid advancement of AI has often outpaced the development of legal frameworks and regulations surrounding its use. This lack of clear guidelines creates uncertainties and risks regarding AI development and deployment. Governments and regulatory bodies must proactively develop legal frameworks that address the unique challenges posed by AI. These frameworks should encompass issues such as privacy, accountability, transparency, and ethical considerations. Collaborative efforts between policymakers, industry experts, and researchers are essential to strike the right balance between innovation and responsible regulation.
5.2 International cooperation
AI is a global phenomenon, and its impact knows no boundaries. To address the challenges associated with AI, international cooperation is crucial. Collaboration between countries can help facilitate knowledge sharing, harmonize regulations, and establish global standards for AI development and deployment. By working together, countries can ensure that AI technologies are developed and used in a manner that respects universal values, ethical principles, and human rights. Encouraging international dialogue and cooperation can promote the responsible and beneficial use of AI on a global scale.
5.3 Governance models for AI
The complex nature of AI necessitates adequate governance and oversight. Establishing governance models for AI is crucial to ensure the responsible development, deployment, and monitoring of AI systems. These models should consider interdisciplinary perspectives, involving experts from various fields like ethics, law, technology, and social sciences. Effective governance frameworks should incorporate stakeholder engagement, transparency, and accountability mechanisms. Ongoing evaluation and adaptation of governance models are necessary to keep pace with the rapidly evolving AI landscape.
6. Data and Bias
6.1 Bias in AI training data
AI systems learn from the data they are trained on. However, if the training data contains biases, these biases can be amplified and perpetuated by AI systems. Bias in AI training data can lead to discriminatory outcomes or reinforce existing societal biases. To address this challenge, it is crucial to ensure diverse, representative, and unbiased training datasets. Data collection practices should prioritize inclusivity and diversity, and data pre-processing techniques should be implemented to identify and mitigate biases. Regular audits and evaluations of AI models can help identify and rectify any biases that emerge during the training process.
6.2 Amplification of societal biases
AI systems have the potential to amplify existing societal biases, exacerbating social inequalities and discrimination. This can occur through biased decision-making, algorithmic profiling, or automated decision systems that perpetuate discriminatory practices. To counter this challenge, it is crucial to incorporate diversity and inclusivity in AI development teams and ensure the involvement of various stakeholders from different backgrounds. Review processes, transparency, and accountability mechanisms should be in place to detect and address instances where AI systems amplify societal biases. Ongoing monitoring and updates are necessary to mitigate these risks.
6.3 Transparent and interpretable models
The opaqueness of AI models can hinder their adoption and trustworthiness. Transparent and interpretable models are essential for understanding AI decision-making processes and ensuring accountability. Researchers and developers should prioritize the development of models that are explainable and understandable to users. Techniques like explainable AI and model interpretability can help shed light on how AI systems arrive at their conclusions. Transparent models can not only assist users in understanding and trusting AI systems, but they can also help uncover biases and identify potential errors or limitations.
7. Long-Term AI Safety
7.1 Superintelligence risks
The potential development of superintelligent AI systems brings both promises and risks. Superintelligent systems surpass human capabilities and may become difficult to control or understand fully. Ensuring the long-term safety of AI requires preemptive measures to mitigate risks associated with superintelligence. Research on AI alignment, value alignment, and control mechanisms is crucial to prevent any unintended consequences or catastrophic scenarios. Ethical considerations and international collaboration can play a pivotal role in establishing guidelines and safeguards to prevent the misuse or unintended consequences of superintelligent AI.
7.2 Control problem and AI alignment
The control problem refers to the challenge of aligning AI system behavior with human values and ensuring human oversight and control. AI systems should follow human intentions and act ethically and responsibly. Achieving AI alignment requires addressing multiple challenges, including value specification, interpretability, and enforcing system boundaries. Researchers and policymakers should focus on developing AI systems that are trainable, accountable, and capable of collaboratively working with humans. Interdisciplinary collaborations, ethical considerations, and continuous monitoring are essential to tackle the control problem and ensure AI behaves in a manner aligned with human values.
7.3 Value-loading dilemma
Value loading refers to the challenge of encoding human values into AI systems. Determining which values to prioritize and how to transfer those values to AI systems is a complex task. The value-loading dilemma arises when different stakeholders hold divergent values, creating conflicts in AI system behavior. Addressing this challenge requires engaging in ethical debates, reaching consensus on common values, and establishing frameworks that allow for value adaptation and customization. Open discussions, public engagement, and interdisciplinary research are key to resolving the value-loading dilemma and ensuring AI systems align with societal values.
8. Resource Allocation
8.1 Accessibility and affordability
Ensuring AI accessibility and affordability are crucial to prevent technology disparities and promote equitable benefits. AI deployment should not be limited to the privileged few. Efforts should be made to bridge the digital divide by making AI technologies accessible to individuals and communities across diverse socioeconomic backgrounds. This includes investing in infrastructure, reducing costs, and promoting education and training programs to enable broad participation and utilization of AI technologies. By improving accessibility and affordability, AI can contribute to narrowing existing societal gaps and promoting inclusive growth.
8.2 Distribution of AI benefits
The benefits and advantages of AI should be distributed equitably to avoid exacerbating existing social inequalities. Without proper measures, AI deployment can concentrate wealth and power in the hands of a few, leading to societal imbalances. To address this challenge, policymakers and stakeholders should channel resources towards AI initiatives that prioritize social impact and benefit everyone. Regular assessments of AI deployment’s social implications, coupled with targeted policies, can help ensure that the benefits of AI are distributed inclusively and contribute to societal progress.
8.3 AI governance funding
The development and maintenance of effective AI governance mechanisms require adequate funding. Allocating resources and funding to AI governance initiatives is essential to establish regulatory frameworks, oversight mechanisms, and research programs that address the unique challenges of AI. Governments, international organizations, and private entities should invest in AI governance funding to support ethical research, policy development, and public awareness campaigns. Sustainable funding models can help foster responsible AI development, governance, and ensure that AI technologies are deployed in a manner that benefits society at large.
9. Public Perception and Education
9.1 Ethical considerations
Public perception of AI is greatly influenced by ethical concerns and considerations. Addressing ethical challenges surrounding AI is vital to build public trust and understanding. Ethical principles should guide AI development and ensure accountability, fairness, and transparency. Engaging in public dialogue and education initiatives that highlight ethical frameworks, risks, and regulations can help dispel fears and misconceptions, fostering a more informed and positive perception of AI technologies.
9.2 Misunderstanding and fear
Misunderstandings and fear often arise from misinformation and exaggerated portrayals of AI in popular culture. Addressing these perceptions requires educational efforts that demystify AI, provide accurate information, and highlight the potential benefits. Promoting media literacy, public debates, and collaborative initiatives between academia, industry, and policymakers can help bridge the knowledge gap and dispel unwarranted fears surrounding AI. Open communication and dialogue can alleviate concerns and facilitate a more nuanced understanding of AI’s capabilities and limitations.
9.3 Public awareness and engagement
Raising public awareness and fostering engagement are crucial for responsible AI development and governance. Public stakeholders should be empowered and involved in AI discussions and decision-making processes. Governments, organizations, and educational institutions should invest in awareness campaigns, education programs, and community engagement initiatives to increase understanding and participation. By involving the public in AI-related discussions, their perspectives can be incorporated, ensuring that AI technologies are developed and governed in a manner that aligns with societal values and interests.
10. Collaboration and Interdisciplinary Research
10.1 Cross-disciplinary expertise
Addressing the multifaceted challenges of AI requires cross-disciplinary expertise and collaboration. Combining knowledge from various fields, including computer science, ethics, sociology, law, and psychology, can help navigate the complex landscape of AI development and deployment. Establishing interdisciplinary research teams, organizing conferences, and fostering collaborations between academia, industry, and policymakers can facilitate the exchange of ideas and ensure comprehensive approaches to AI challenges.
10.2 Knowledge sharing and cooperation
Knowledge sharing and cooperation are instrumental in overcoming AI challenges. Open dialogue and the sharing of research findings, best practices, and lessons learned can accelerate progress. Researchers, developers, and policymakers should actively participate in knowledge-sharing platforms, conferences, and collaborations to collectively advance AI development, governance, and safety. International cooperation and the establishment of networks that facilitate knowledge exchange can foster a global community working towards responsible and beneficial AI technologies.
10.3 Open-source AI development
Open-source AI development can promote transparency, collaboration, and innovation. By making AI software and research publicly available, researchers and developers can benefit from collective expertise and contributions. Open-source AI initiatives not only encourage collaboration but also facilitate the identification and mitigation of potential risks and biases. Governments, organizations, and funding agencies should support and promote open-source AI development, facilitating inclusive participation and collective efforts towards responsible AI systems.
In conclusion, while AI presents immense opportunities for innovation and progress, there are numerous challenges that need to be overcome. Technical limits, ethics, human-AI interaction, robustness and security, regulation and governance, data and bias, long-term AI safety, resource allocation, public perception and education, and collaboration and interdisciplinary research are key areas that require focused attention. By addressing these challenges in a responsible and inclusive manner, we can harness the benefits of AI while ensuring that it is developed and deployed for the betterment of society as a whole.